A real-time non-tracking monitoring video remnant detection method
A technology of monitoring video and detection methods, which is applied in the field of image processing, and can solve problems such as not taking into account changes in objects and not being able to recognize them
- Summary
- Abstract
- Description
- Claims
- Application Information
AI Technical Summary
Problems solved by technology
Method used
Image
Examples
Embodiment 1
[0092] image 3 The detection results of our own test video are given, and the scene is a corridor. image 3 It is an experiment done when there is light, and compared with literature [6] and literature [16]. There are two remnants in the video, which are bags and cartons; image 3 It can be seen from the results that the method in this paper can better exclude the area generated by the light than the other two methods when there is light, and can accurately detect the remnants.
Embodiment 2
[0094] We also apply the method in this paper to the i-LIDS [15] dataset and the ABODA [6] dataset for carryover detection. The scenes in the i-LIDS dataset are divided into three levels: simple, medium, and complex with the change of light and traffic. These three scenarios are tested separately in this paper. And compared with literature [6] and literature [16]. Figure 4 The difficulty of target detection from top to bottom: simple (AB-Easy), medium (AB-Medium), complex (AB-Hard). In the video AB-Easy, the objects left behind in the scene are relatively large, which is relatively easy to detect; in the video AB-Medium, the objects are small and relatively far away, and the detection difficulty is moderate; in the video AB-Hard, the scene is more complicated, objects are farther away, and people More, the detection is more difficult. Figure 4 (a), (4)b, and (4)c are the frames when the remnants actually occurred in the three videos, respectively, and the blue font in the...
PUM
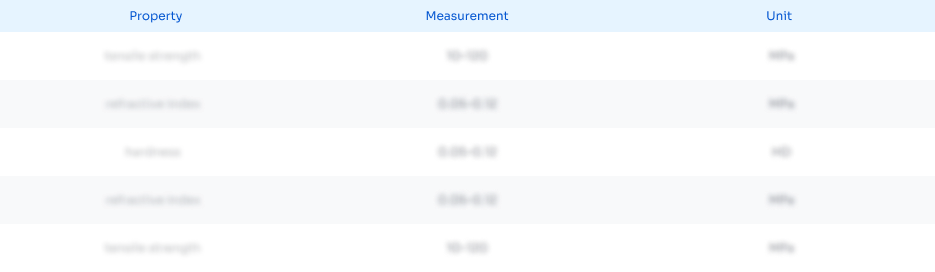
Abstract
Description
Claims
Application Information
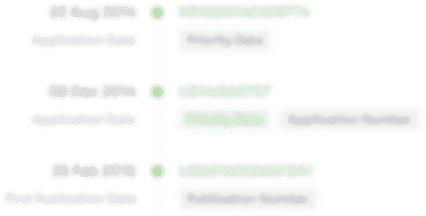
- R&D Engineer
- R&D Manager
- IP Professional
- Industry Leading Data Capabilities
- Powerful AI technology
- Patent DNA Extraction
Browse by: Latest US Patents, China's latest patents, Technical Efficacy Thesaurus, Application Domain, Technology Topic, Popular Technical Reports.
© 2024 PatSnap. All rights reserved.Legal|Privacy policy|Modern Slavery Act Transparency Statement|Sitemap|About US| Contact US: help@patsnap.com