A remote sensing image multi-label retrieval method and system based on a full convolutional neural network
A convolutional neural network, remote sensing image technology, applied in the field of remote sensing image multi-label retrieval methods and systems, can solve the problem of ignoring image multi-category information and other problems
- Summary
- Abstract
- Description
- Claims
- Application Information
AI Technical Summary
Problems solved by technology
Method used
Image
Examples
Embodiment Construction
[0044] For the technical solution of the present invention in detail, refer to figure 1 , the specific description of the embodiment process is provided as follows:
[0045] The multi-label retrieval technology scheme of remote sensing images based on fully convolutional neural network proposed by the present invention first divides remote sensing images into blocks to construct an image library for retrieval, and then constructs an FCN model based on a pre-trained convolutional neural network model and utilizes the training The network training is completed, and then the local features of the image are extracted from each convolutional layer based on the segmentation result map of the FCN and encoded to obtain the feature vector for retrieval. Finally, the gradual progression from coarse to fine is realized according to the preset similarity measurement criteria. retrieve and return similar images.
[0046] see figure 1 , the specific implementation of the flow process of t...
PUM
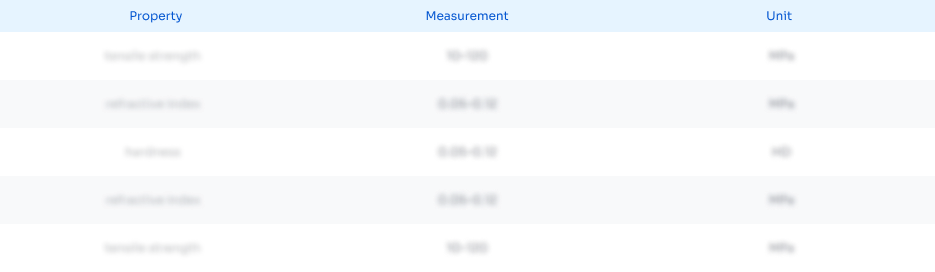
Abstract
Description
Claims
Application Information
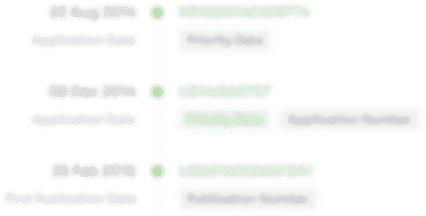
- R&D Engineer
- R&D Manager
- IP Professional
- Industry Leading Data Capabilities
- Powerful AI technology
- Patent DNA Extraction
Browse by: Latest US Patents, China's latest patents, Technical Efficacy Thesaurus, Application Domain, Technology Topic, Popular Technical Reports.
© 2024 PatSnap. All rights reserved.Legal|Privacy policy|Modern Slavery Act Transparency Statement|Sitemap|About US| Contact US: help@patsnap.com