An Image Classification Method Based on Dimension Transformation of Observation Matrix
A technology of observation matrix and classification method, applied in instrument, calculation, character and pattern recognition, etc., can solve the problems of unfavorable storage and transmission, increase of calculation amount, low model parameter adjustment training and use efficiency, etc., to improve the efficiency of the model. Effect
- Summary
- Abstract
- Description
- Claims
- Application Information
AI Technical Summary
Problems solved by technology
Method used
Image
Examples
Embodiment Construction
[0045] The present invention will be further described below in conjunction with the accompanying drawings and embodiments.
[0046] Such as figure 1 As shown, this embodiment provides an image classification method based on the transformation dimension of the observation matrix, including the following steps:
[0047] (1) Use perceptual compression to sparsely encode images to obtain a data set composed of low-dimensional images. Divide the data set containing labels into training set and test set, and the division ratio is 8:2.
[0048] Methods for sparsely coding images using perceptual compression include sparse representation of images, image compression sampling, and image reconstruction.
[0049] (1-1) Image sparse representation is:
[0050] Express the original signal x on a set of sparse basis Ψ:
[0051] x=Ψs
[0052] Among them, x is the original signal, its size is N×1, Ψ is a set of sparse basis, and s is the sparse coefficient.
[0053] s is an N×1 column v...
PUM
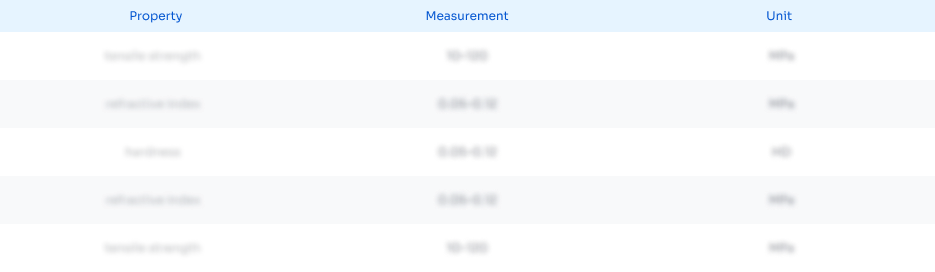
Abstract
Description
Claims
Application Information
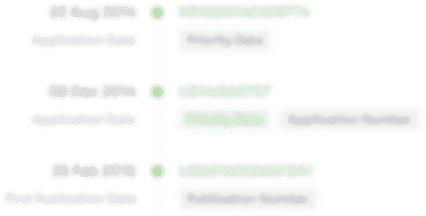
- R&D Engineer
- R&D Manager
- IP Professional
- Industry Leading Data Capabilities
- Powerful AI technology
- Patent DNA Extraction
Browse by: Latest US Patents, China's latest patents, Technical Efficacy Thesaurus, Application Domain, Technology Topic, Popular Technical Reports.
© 2024 PatSnap. All rights reserved.Legal|Privacy policy|Modern Slavery Act Transparency Statement|Sitemap|About US| Contact US: help@patsnap.com