An image segmentation method based on appearance dictionary learning and shape sparse representation
A sparse representation and dictionary learning technology, applied in the field of image processing, can solve problems such as single prior, low organ accuracy and reliability, and difficult processing of abnormal data, and achieve accurate peak adjustment and precise lung segmentation results Effect
- Summary
- Abstract
- Description
- Claims
- Application Information
AI Technical Summary
Problems solved by technology
Method used
Image
Examples
Embodiment Construction
[0010] The present invention will be further described below in conjunction with the accompanying drawings.
[0011] The lung segmentation method of the present invention is intended to make full and reasonable use of prior knowledge of appearance and prior knowledge of shape. like figure 1 It is a slice image of lung low-dose CT, where (a) and (c) are enlarged images of left and right lungs. The specific division method is as follows:
[0012] 1. Filter and denoise the original image. The lungs are manually marked as the gold standard for segmentation, that is, the exact lung area in the image, so that the segmentation accuracy of the segmentation method of the present invention can be finally compared. Transform the calibrated gold standard into the form of a 3D grid. The relationship between each 3D mesh is fixed and has the same number of edges and vertices. For the image to be segmented, threshold segmentation and morphological operations are used to obtain a binary ...
PUM
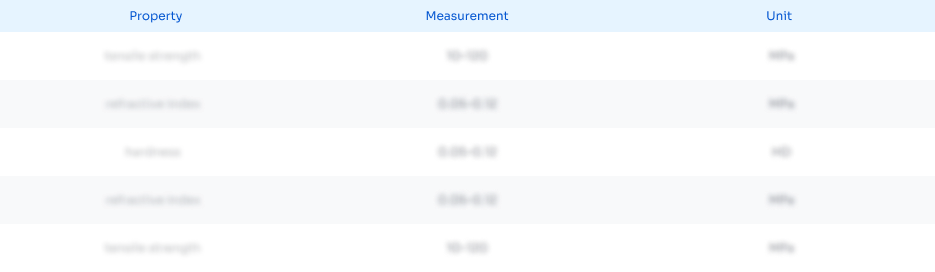
Abstract
Description
Claims
Application Information
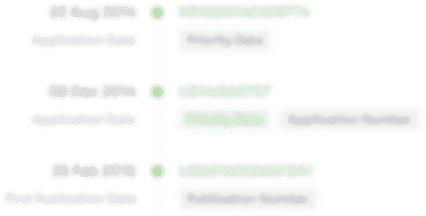
- R&D
- Intellectual Property
- Life Sciences
- Materials
- Tech Scout
- Unparalleled Data Quality
- Higher Quality Content
- 60% Fewer Hallucinations
Browse by: Latest US Patents, China's latest patents, Technical Efficacy Thesaurus, Application Domain, Technology Topic, Popular Technical Reports.
© 2025 PatSnap. All rights reserved.Legal|Privacy policy|Modern Slavery Act Transparency Statement|Sitemap|About US| Contact US: help@patsnap.com