A Bayesian optimization method based on a sequential Monte Carlo method
A technique of sequential Monte Carlo, optimization methods, applied in directions based on specific mathematical models, computational models, calculations, etc.
- Summary
- Abstract
- Description
- Claims
- Application Information
AI Technical Summary
Problems solved by technology
Method used
Image
Examples
Embodiment Construction
[0065] The technical solution of the present invention will be further described below in conjunction with the accompanying drawings.
[0066] Such as figure 1 As shown, a Bayesian optimization method based on sequential Monte Carlo method, including the following steps:
[0067] S1. Establish the objective function and obtain the posterior distribution of the objective function; the specific implementation method is:
[0068] For a d-dimensional random variable X, the multivariate Student-t distribution is given by:
[0069]
[0070] Among them, M d (x, μ, ∑) = (x-μ) T ∑ -1 (x-μ); M d (x, μ, ∑) represents the squared Mahalanobis distance from x to the mean μ with respect to the covariance matrix Σ; Explanatory; the additional parameter ν>2 describes the degrees of freedom of the distribution and acts as a smoothing factor when changing; when ν→∞, the Student-t distribution converges to a Gaussian distribution with the same mean and covariance matrix;
[0071] The ob...
PUM
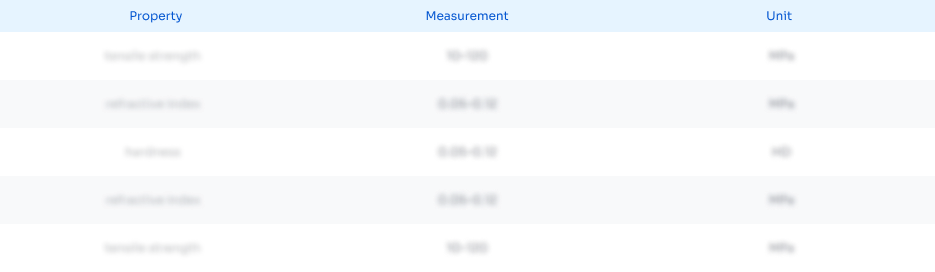
Abstract
Description
Claims
Application Information
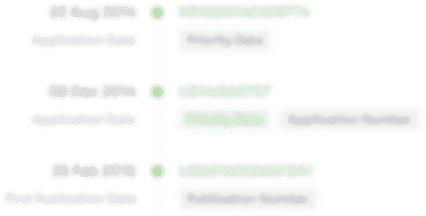
- R&D
- Intellectual Property
- Life Sciences
- Materials
- Tech Scout
- Unparalleled Data Quality
- Higher Quality Content
- 60% Fewer Hallucinations
Browse by: Latest US Patents, China's latest patents, Technical Efficacy Thesaurus, Application Domain, Technology Topic, Popular Technical Reports.
© 2025 PatSnap. All rights reserved.Legal|Privacy policy|Modern Slavery Act Transparency Statement|Sitemap|About US| Contact US: help@patsnap.com