Unsupervised domain adaptive image classification method based on conditional generative adversarial network
A technology of conditional generation and classification methods, applied in biological neural network models, computer components, instruments, etc., can solve problems such as the limitations of classification tasks, achieve considerable use value, improve classification accuracy, and improve domain adaptability.
- Summary
- Abstract
- Description
- Claims
- Application Information
AI Technical Summary
Problems solved by technology
Method used
Image
Examples
Embodiment Construction
[0042] The technical solution of the present invention will be described in detail below in conjunction with the accompanying drawings.
[0043] Such as figure 1 As shown, the present invention proposes an unsupervised domain adaptive image classification method based on a conditional generation confrontation network. The core step of the present invention is to construct a conditional confrontation image generation network and combine the labeled source domain images to effectively use unlabeled targets. The domain image is trained, and the description of the specific implementation mainly focuses on step 2.
[0044] Step 1. Image preprocessing
[0045] The quality of the image has a direct impact on the realization of the algorithm and the classification effect. Normalizing the image is a way to simplify the calculation, which is of great significance for improving the classification accuracy. Given an image sample X, according to the formula img=(X-mean) / std, where mean and std...
PUM
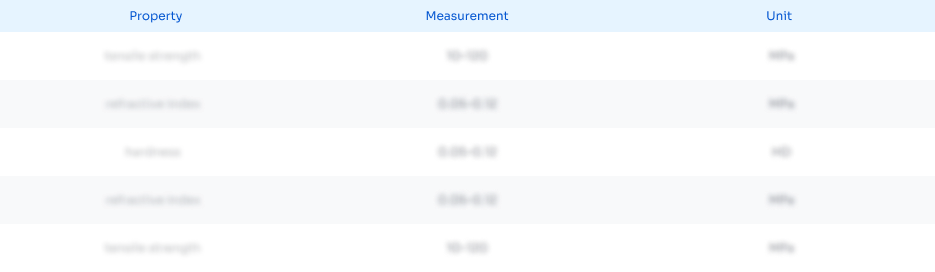
Abstract
Description
Claims
Application Information
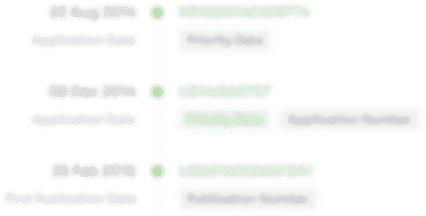
- R&D Engineer
- R&D Manager
- IP Professional
- Industry Leading Data Capabilities
- Powerful AI technology
- Patent DNA Extraction
Browse by: Latest US Patents, China's latest patents, Technical Efficacy Thesaurus, Application Domain, Technology Topic, Popular Technical Reports.
© 2024 PatSnap. All rights reserved.Legal|Privacy policy|Modern Slavery Act Transparency Statement|Sitemap|About US| Contact US: help@patsnap.com