Form data privacy protection method fusing differential privacy GAN model and PATE model
A technology of differential privacy and tabular data, applied in the field of privacy protection, it can solve the problems of exposing the privacy of individual samples and generating great influence of the model.
- Summary
- Abstract
- Description
- Claims
- Application Information
AI Technical Summary
Problems solved by technology
Method used
Image
Examples
Embodiment Construction
[0045] The technical solution of the present invention will be specifically described below in conjunction with the accompanying drawings.
[0046] The present invention provides a tabular data privacy protection method that integrates differential privacy GAN and PATE models, comprising the following steps:
[0047] Step S1, using the original tabular data to train the differentially private generative model; wherein, the differentially private generative model training process includes two parts: generative adversarial network training and discriminative model adding noise perturbation, as follows:
[0048] Step S11, selection of an adversarial network:
[0049] The auxiliary classification generation confrontation network uses the original table data and labels as input. In the discriminative model part, it not only distinguishes the true and false data, but also predicts the category of the data. Therefore, the auxiliary classification generation confrontation network is s...
PUM
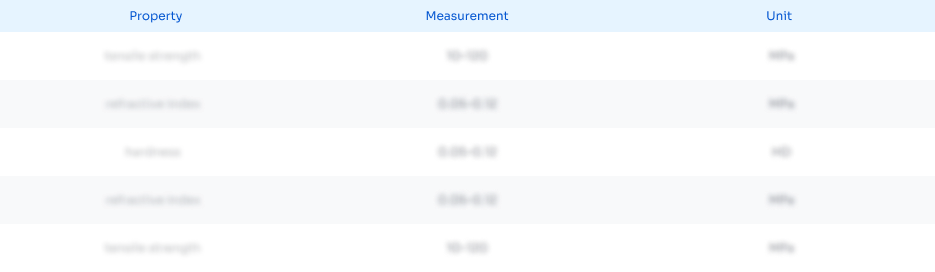
Abstract
Description
Claims
Application Information
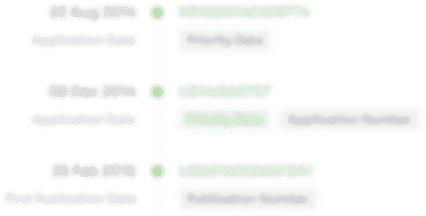
- R&D Engineer
- R&D Manager
- IP Professional
- Industry Leading Data Capabilities
- Powerful AI technology
- Patent DNA Extraction
Browse by: Latest US Patents, China's latest patents, Technical Efficacy Thesaurus, Application Domain, Technology Topic, Popular Technical Reports.
© 2024 PatSnap. All rights reserved.Legal|Privacy policy|Modern Slavery Act Transparency Statement|Sitemap|About US| Contact US: help@patsnap.com