Hyperspectral image semi-supervised classification method based on comprehensive confidence
A hyperspectral image and classification method technology, applied in the field of hyperspectral image semi-supervised classification based on comprehensive confidence, can solve the problems of hyperspectral image classification ability to be improved, weak class boundary distinction ability, rough classification results of hyperspectral image, etc.
- Summary
- Abstract
- Description
- Claims
- Application Information
AI Technical Summary
Problems solved by technology
Method used
Image
Examples
Embodiment
[0059] This embodiment provides a method for semi-supervised classification of hyperspectral images based on comprehensive confidence, the process of the method is as follows figure 1 shown, including the following steps:
[0060] S1. Read in the three-dimensional hyperspectral image cube H(m,n,b), where m and n represent the spatial pixel position, and b represents the spectral band position;
[0061] S2. Calculate the correlation coefficient of the sample mean value between hyperspectral data pixels, which is used to construct the graph weight matrix W. The weight value can measure the similarity between pixels. The calculation method is as follows:
[0062]
[0063] Among them, v i Represents the data feature of the i-th pixel, v a Represents the mean value of data features of all pixels, w ij Is the element in the graph weight matrix W, representing the similarity weight value of pixel i and pixel j;
[0064] S3. Set the similarity weight value of each pixel in the ...
PUM
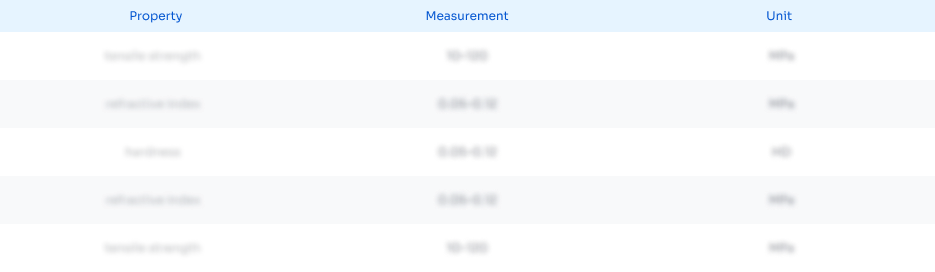
Abstract
Description
Claims
Application Information
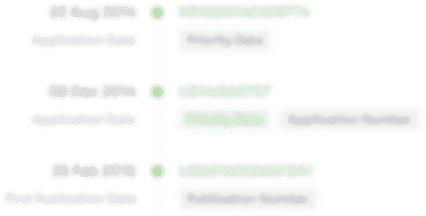
- Generate Ideas
- Intellectual Property
- Life Sciences
- Materials
- Tech Scout
- Unparalleled Data Quality
- Higher Quality Content
- 60% Fewer Hallucinations
Browse by: Latest US Patents, China's latest patents, Technical Efficacy Thesaurus, Application Domain, Technology Topic, Popular Technical Reports.
© 2025 PatSnap. All rights reserved.Legal|Privacy policy|Modern Slavery Act Transparency Statement|Sitemap|About US| Contact US: help@patsnap.com