A shape filling type reading understanding analysis model and method based on reinforcement learning
A technology of reinforcement learning and reading comprehension, applied in the field of machine learning, can solve problems such as high computational complexity, low computational efficiency, and low accuracy rate, and achieve the effect of improving computational efficiency, improving accuracy, and reducing the amount of computation
- Summary
- Abstract
- Description
- Claims
- Application Information
AI Technical Summary
Problems solved by technology
Method used
Image
Examples
Embodiment Construction
[0048] The implementation of the present invention is described below through specific examples and in conjunction with the accompanying drawings, and those skilled in the art can easily understand other advantages and effects of the present invention from the content disclosed in this specification. The present invention can also be implemented or applied through other different specific examples, and various modifications and changes can be made to the details in this specification based on different viewpoints and applications without departing from the spirit of the present invention.
[0049] Before introducing the present invention, the abbreviations and key terms involved in the present invention are defined as follows:
[0050] Language Model: Language Model (Language Model) is a model used to calculate the probability of a sentence, that is, the probability P(w 1 ,w 2 ,...,w k ), it is widely used in various natural language processing problems such as machine readi...
PUM
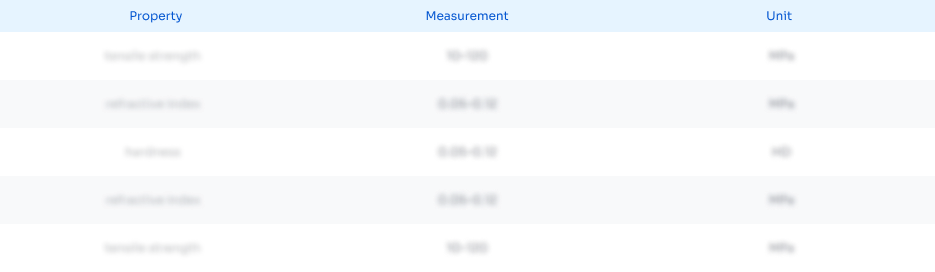
Abstract
Description
Claims
Application Information
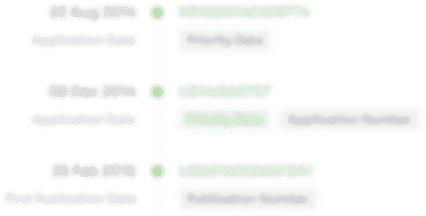
- R&D Engineer
- R&D Manager
- IP Professional
- Industry Leading Data Capabilities
- Powerful AI technology
- Patent DNA Extraction
Browse by: Latest US Patents, China's latest patents, Technical Efficacy Thesaurus, Application Domain, Technology Topic.
© 2024 PatSnap. All rights reserved.Legal|Privacy policy|Modern Slavery Act Transparency Statement|Sitemap