Image feature extraction method based on weighted depth features
An image feature extraction and depth feature technology, applied in the field of image processing, can solve the problems of complex calculation, poor versatility, low feature robustness, etc., and achieve the effects of good robustness, easy implementation and good versatility
- Summary
- Abstract
- Description
- Claims
- Application Information
AI Technical Summary
Problems solved by technology
Method used
Image
Examples
Embodiment 1
[0049] Such as figure 1 Shown, a kind of image feature extraction method based on weighted depth feature, described method comprises the following steps:
[0050] S1: Select the existing network model VGG16 image classification network to pre-train on the ImageNet image data set, and remove the softmax layer and fully connected layer in the network model after pre-training to obtain the final VGG16 image classification network model; (The existing model is a classification network model or a location detection network model, selected according to a specific image task. The image data set used for pre-training is an existing common data set or based on an image data set to be extracted.)
[0051] S2: First, directly input the image to be extracted into the VGG16 image classification network model without the softmax layer and fully connected layer for forward calculation, and then extract the convolutional layer in front of all pooling layers in the VGG16 image classification n...
Embodiment 2
[0098] A method for extracting image features based on weighted depth features, said method comprising the following steps:
[0099] S1: In this embodiment, the network model ResNet is selected to perform pre-training on the coco image data set, and the softmax layer and the fully connected layer in the network model after the pre-training are completed are removed to obtain the final ResNet image classification network model; (described The existing model is a classification network model or a location detection network model, which is selected according to the specific image task. The image data set used for pre-training is the existing common data set or based on the image data set to be extracted.)
[0100] S2: First, input the image to be extracted directly into the ResNet image classification network model without the softmax layer and fully connected layer for forward calculation, and then extract the convolutional layer in front of all pooling layers in the ResNet image...
PUM
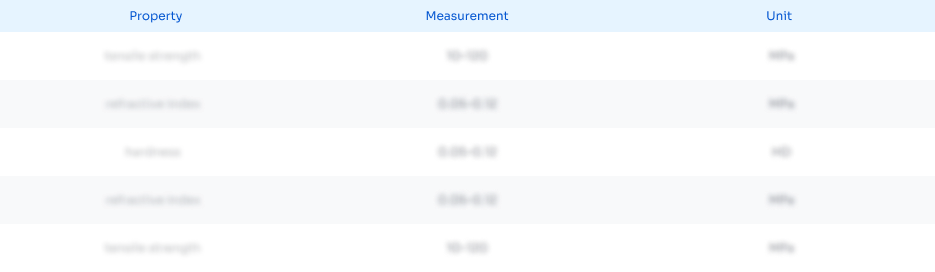
Abstract
Description
Claims
Application Information
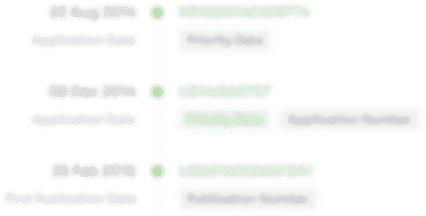
- R&D Engineer
- R&D Manager
- IP Professional
- Industry Leading Data Capabilities
- Powerful AI technology
- Patent DNA Extraction
Browse by: Latest US Patents, China's latest patents, Technical Efficacy Thesaurus, Application Domain, Technology Topic, Popular Technical Reports.
© 2024 PatSnap. All rights reserved.Legal|Privacy policy|Modern Slavery Act Transparency Statement|Sitemap|About US| Contact US: help@patsnap.com