Robot navigation method based on pre-processing layer and deep enhanced learning
A technology of reinforcement learning and preprocessing layer, applied in the field of robot navigation, can solve problems such as poor generalization performance, and achieve the effect of excellent transfer performance
- Summary
- Abstract
- Description
- Claims
- Application Information
AI Technical Summary
Problems solved by technology
Method used
Image
Examples
specific Embodiment 1
[0085] For the navigation method of the present invention, in combination with Figure 2 to Figure 12 The present invention is further described in specific embodiments in practical application and calculation process:
[0086] build as image 3 In the virtual training environment shown, in the virtual training environment, the virtual sensor uses an RGB camera, the virtual robot uses a virtual TURTLEBOT model, the virtual environment uses GAZEBO (simulation robot software), and the communication layer uses the ROS multi-computer communication method. The settings are printed with The square of the number 9 is an obstacle, the number 2 printed on the wall is set as the target, and the number 4 and 8 are the left and right position information respectively.
[0087] Considering that in the training process there are such Figure 4 The difference between the virtual environment shown and the real environment is too large (the gray value matrix is too different), and when deep...
specific Embodiment 2
[0113] Figure 10-Figure 12 Another specific embodiment of the navigation method of the present invention is specifically applied.
Embodiment 2
[0114] Embodiment 2 is a virtual training environment based on embodiment 1. The same virtual sensor has adopted an RGB camera, and the virtual robot has adopted a virtual TURTLEBOT model. The square with the "fire" picture is set as an obstacle, the rescued person printed on the white paper is set as the target, and the real robot is set as the rescuer.
[0115] Adopt the same method as embodiment 1 to train the rescue robot, the observation statistics are obtained as follows Figure 10 The number of training iteration steps of the virtual robot navigation task in the virtual environment is shown. It can be seen that with the increase of training rounds, the number of steps for the robot to complete the task gradually decreases until the deep reinforcement learning converges at about 120,000 rounds.
[0116] Migrate the results of deep reinforcement learning in the virtual environment to the real environment. Specifically, after the training in the virtual environment is comp...
PUM
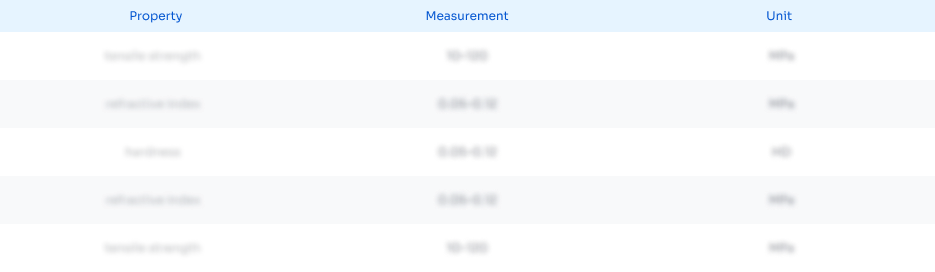
Abstract
Description
Claims
Application Information
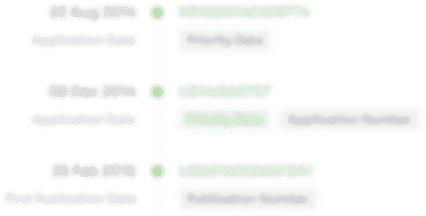
- R&D Engineer
- R&D Manager
- IP Professional
- Industry Leading Data Capabilities
- Powerful AI technology
- Patent DNA Extraction
Browse by: Latest US Patents, China's latest patents, Technical Efficacy Thesaurus, Application Domain, Technology Topic, Popular Technical Reports.
© 2024 PatSnap. All rights reserved.Legal|Privacy policy|Modern Slavery Act Transparency Statement|Sitemap|About US| Contact US: help@patsnap.com