A building contour automatic extraction algorithm based on a convolutional neural network and polygon regularization
A neural network, automatic extraction technology, applied in computing, image analysis, instruments, etc., can solve problems such as inability to truly automate, and achieve the effect of reducing workload and strong robustness
- Summary
- Abstract
- Description
- Claims
- Application Information
AI Technical Summary
Problems solved by technology
Method used
Image
Examples
Embodiment
[0051] see figure 1 and figure 2 , the present invention uses a multi-scale fusion full convolutional neural network (Multi-scaleAggregation Full Convolutional Neural Network, MA-FCN) to learn building features in high-resolution remote sensing images, and then performs pixel-level prediction of building coverage in remote sensing images. In order to train the neural network model, it is first necessary to obtain training samples, attached figure 1 Shows the process of building a training sample library. Firstly, the remote sensing images are cropped and resampled to obtain an image range with appropriate resolution and building coverage data. The corresponding building vector data within the image extent is then rasterized to match the image resolution. Finally, the remote sensing images and corresponding label data are divided into sample blocks of appropriate size (such as 256×256 pixels, or 512×512 pixels) in combination with factors such as computer performance and th...
PUM
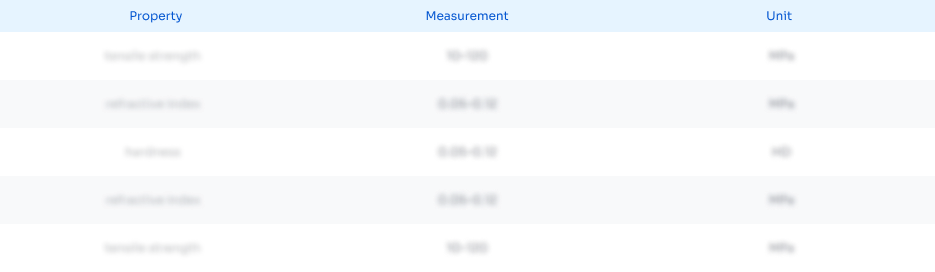
Abstract
Description
Claims
Application Information
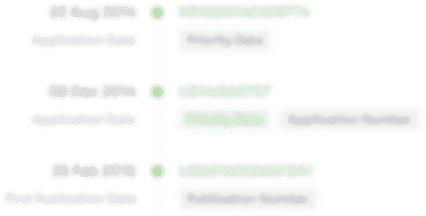
- R&D
- Intellectual Property
- Life Sciences
- Materials
- Tech Scout
- Unparalleled Data Quality
- Higher Quality Content
- 60% Fewer Hallucinations
Browse by: Latest US Patents, China's latest patents, Technical Efficacy Thesaurus, Application Domain, Technology Topic, Popular Technical Reports.
© 2025 PatSnap. All rights reserved.Legal|Privacy policy|Modern Slavery Act Transparency Statement|Sitemap|About US| Contact US: help@patsnap.com