Rolling bearing fault diagnosis method based on improved multi-scale amplitude perceived permutation entropy
A rolling bearing and fault diagnosis technology, which is applied in the testing of mechanical components, testing of machine/structural components, measuring devices, etc., can solve problems such as low accuracy of fault identification, weak separability, and insufficient analysis of fault severity , to achieve the effects of good separability, strong fault description ability, and good fault severity description ability
- Summary
- Abstract
- Description
- Claims
- Application Information
AI Technical Summary
Problems solved by technology
Method used
Image
Examples
specific Embodiment approach 1
[0025] Specific implementation mode one: the following combination figure 1 This embodiment will be specifically described. This embodiment is based on the rolling bearing fault diagnosis method based on the improved multi-scale amplitude permutation entropy, including the following steps:
[0026] Step 1: Obtain known vibration signals of rolling bearings under different types of faults and different fault degrees, and form sample sets of vibration signals of rolling bearings under different types of faults and different fault degrees;
[0027] Step 2: For each vibration signal in the sample set, decompose the inherent time scale to obtain a series of inherent rotation PR components, and select the optimal PR component for subsequent feature extraction;
[0028] Step 3: Using the improved multi-scale amplitude perception permutation entropy to extract the characteristics of the rolling bearing vibration signals contained in the optimal PR component at different time scales, ...
Embodiment
[0039]The present invention selects the rolling bearing fault data set provided by the Bearing Data Center of Western Reserve University in the United States to carry out experimental verification on the proposed fault diagnosis method. The experiment takes SKF bearings as the research object. The data set collects vibration signals of bearings in four states: normal (NM), inner ring fault (IR), outer ring fault (OR) and ball fault (B) through the acceleration sensor. The sampling frequency is 12KHz; for the three types of faults, three different fault severities with fault diameters of 7mils, 14mils and 21mils were respectively selected for data acquisition. The time-domain waveforms of rolling bearing vibration signals with different fault severities under 0 load are as follows: figure 2 and image 3 As shown in , it can be seen that the changes in the type of fault and the severity of the fault are related to the changes in the amplitude and frequency of its vibration sig...
specific Embodiment approach 2
[0070] Specific embodiment 2: This embodiment is a further description of specific embodiment 1. The difference between this embodiment and specific embodiment 1 is that in the step 2, set X t For the known signal to be analyzed, define is the baseline extraction operator, able to extract X t medium baseline signal And get the corresponding intrinsic rotation component Signal X t Decomposed into
[0071]
[0072] The main steps of the intrinsic time scale decomposition algorithm are as follows:
[0073] Step 21: Suppose {τ k ,k=1,2,...} means signal X t The local extremum of , the default τ 0 = 0;
[0074] Step 22: In the interval [0,τ k ] define L in t and H t , and X t In the interval t∈[0,τ k+2 ], in the continuous extremum interval (τ k ,τ k+1 ] The extracted baseline signal L in t Expressed as:
[0075]
[0076] in,
[0077] In the formula, α is a linear scaling factor, which is used to adjust the amplitude of the extracted intrinsic rotatio...
PUM
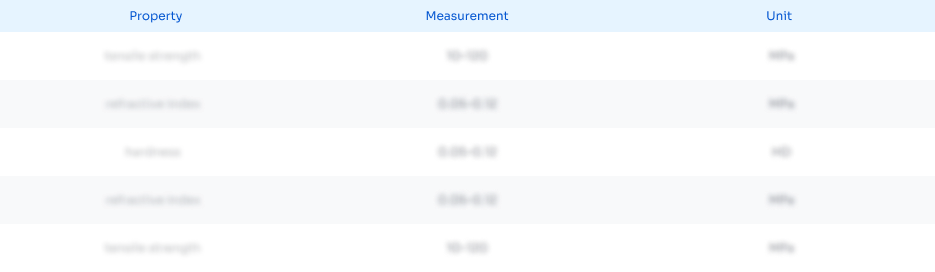
Abstract
Description
Claims
Application Information
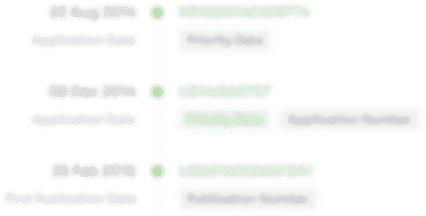
- Generate Ideas
- Intellectual Property
- Life Sciences
- Materials
- Tech Scout
- Unparalleled Data Quality
- Higher Quality Content
- 60% Fewer Hallucinations
Browse by: Latest US Patents, China's latest patents, Technical Efficacy Thesaurus, Application Domain, Technology Topic, Popular Technical Reports.
© 2025 PatSnap. All rights reserved.Legal|Privacy policy|Modern Slavery Act Transparency Statement|Sitemap|About US| Contact US: help@patsnap.com