Subway passenger congestion degree prediction method adopting a resampling recurrent neural network
A recursive neural network and prediction method technology, applied in the field of subway passenger congestion prediction, can solve the problem of difficult subway congestion prediction by models, and achieve the effect of uniform sampling
- Summary
- Abstract
- Description
- Claims
- Application Information
AI Technical Summary
Problems solved by technology
Method used
Image
Examples
Embodiment Construction
[0050] The following are specific embodiments of the present invention and in conjunction with the accompanying drawings, the technical solutions of the present invention are further described, but the present invention is not limited to these embodiments.
[0051] A method for predicting subway congestion using a resampling recurrent neural network, comprising the following steps:
[0052] Step 1: Set the training sample data according to the original data;
[0053] Step 2: Set 4 congestion labels, which are non-crowded, mildly congested, moderately congested, and severely congested. Divide the training sample data obtained in step 1 into 4 sub-sample sets according to the crowding degree label;
[0054] Step 3: Resample the sub-sample set obtained in Step 2, the resampling weight is randomly selected, and set the resampling sequence according to the resampling result;
[0055] Step 4: Input the resampling sequence obtained in Step 3 into the recurrent neural network model ...
PUM
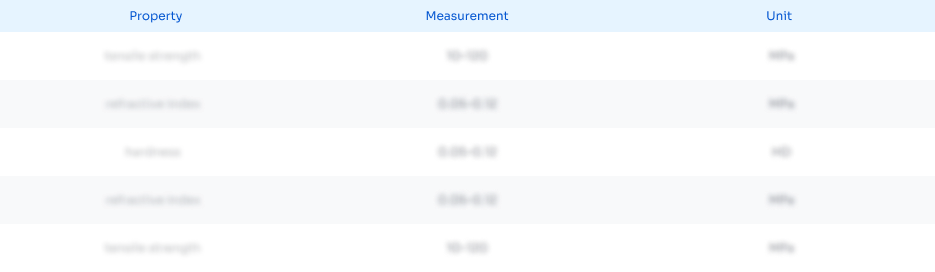
Abstract
Description
Claims
Application Information
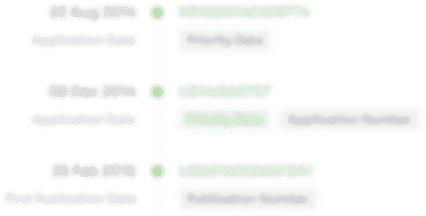
- R&D
- Intellectual Property
- Life Sciences
- Materials
- Tech Scout
- Unparalleled Data Quality
- Higher Quality Content
- 60% Fewer Hallucinations
Browse by: Latest US Patents, China's latest patents, Technical Efficacy Thesaurus, Application Domain, Technology Topic, Popular Technical Reports.
© 2025 PatSnap. All rights reserved.Legal|Privacy policy|Modern Slavery Act Transparency Statement|Sitemap|About US| Contact US: help@patsnap.com