Full-reference and no-reference image quality assessment methods with unified structure
An image quality evaluation and reference image technology, applied in the field of image processing, can solve problems such as difficult generalization ability, ignoring the application of knowledge in the image field, and differences, etc., to achieve labor cost saving, strong practicability, reliability, and strong portability Effect
- Summary
- Abstract
- Description
- Claims
- Application Information
AI Technical Summary
Problems solved by technology
Method used
Image
Examples
Embodiment Construction
[0027] The following embodiments will further illustrate the present invention in conjunction with the accompanying drawings.
[0028] Embodiments of the present invention include the following steps:
[0029] (1) Quality evaluation method data preparation and image preprocessing method
[0030] The input of the no-reference image quality assessment method and the input image preprocessing of the no-reference image quality assessment method are explained in detail below.
[0031]Step 1: Correspond the distorted image in the data set with the average difference subjective score (DMOS) of the reference image and the distorted image and save it in a file. The smaller the value of the average difference subjective score, the better the image quality, for example, the average difference score of the reference image is 0 points. If the dataset provides a Mean Subjective Quality Score (MOS), convert the score to a Difference Subjective Score (DMOS) in the range [0,100]. The specif...
PUM
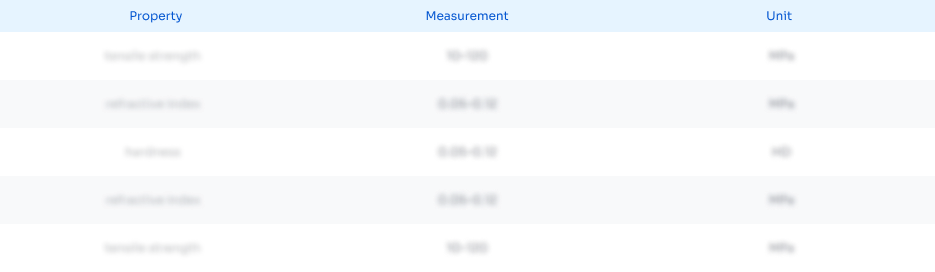
Abstract
Description
Claims
Application Information
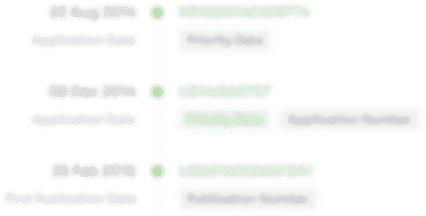
- R&D Engineer
- R&D Manager
- IP Professional
- Industry Leading Data Capabilities
- Powerful AI technology
- Patent DNA Extraction
Browse by: Latest US Patents, China's latest patents, Technical Efficacy Thesaurus, Application Domain, Technology Topic, Popular Technical Reports.
© 2024 PatSnap. All rights reserved.Legal|Privacy policy|Modern Slavery Act Transparency Statement|Sitemap|About US| Contact US: help@patsnap.com