Automatic model compression method based on Q-Learning algorithm
A compression method and model technology, applied in the field of deep learning, to achieve the effects of reduced energy consumption, reduced energy consumption and reasoning time, and efficient embedded reasoning
- Summary
- Abstract
- Description
- Claims
- Application Information
AI Technical Summary
Problems solved by technology
Method used
Image
Examples
Embodiment
[0037] Examples, see figure 1 :
[0038] 1) Build a deep learning environment on the JD Cloud server and NVIDIA Jetson TX2 embedded mobile platform, and select five classic deep neural network models from github for backup, including MobileNet, Inceptionv3, ResNet50, VGG16 and NMT models.
[0039] 2) Analyze and design the state set, action set, and reward function in the Q-Learning algorithm according to the constraints, and complete the algorithm coding and scripting of the model performance test.
[0040] 3) Integrate and modify the codes of different model compression technologies and choose MobileNet to test on NVIDIAJetson TX2, and make a preliminary judgment on the performance of different compression algorithms.
[0041] 4) Transplant the code to the JD Cloud server and set different demand coefficients to select compression algorithms for the five network models and save all the compressed models.
[0042] 5) Transplant all the models before and after compression to...
PUM
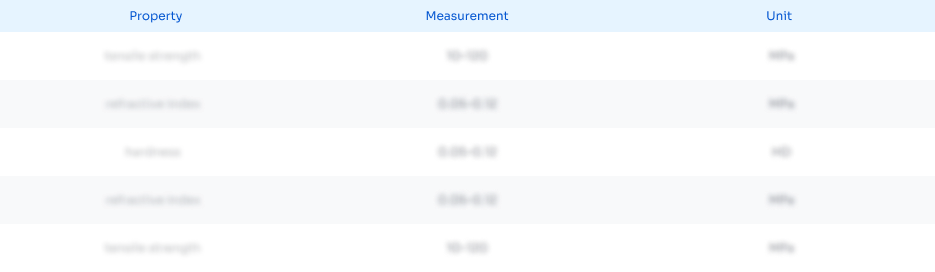
Abstract
Description
Claims
Application Information
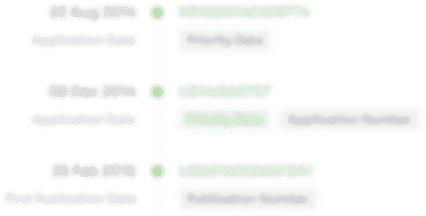
- R&D
- Intellectual Property
- Life Sciences
- Materials
- Tech Scout
- Unparalleled Data Quality
- Higher Quality Content
- 60% Fewer Hallucinations
Browse by: Latest US Patents, China's latest patents, Technical Efficacy Thesaurus, Application Domain, Technology Topic, Popular Technical Reports.
© 2025 PatSnap. All rights reserved.Legal|Privacy policy|Modern Slavery Act Transparency Statement|Sitemap|About US| Contact US: help@patsnap.com