Novel multi-round dialogue natural language understanding model based on BERTCONTEXT
A natural language understanding and model technology, applied in biological neural network models, electrical digital data processing, special data processing applications, etc., can solve the problems of poor NLU task effect, no design of multiple rounds of dialogue NLU, and unequal semantic information. Improved accuracy, reduced risk of overfitting, and improved experience
- Summary
- Abstract
- Description
- Claims
- Application Information
AI Technical Summary
Problems solved by technology
Method used
Image
Examples
Embodiment 1
[0028] figure 2 It is a structural schematic diagram of multi-task based on parameter sharing in an embodiment of the present invention. When multiple tasks are learned together, for one of the tasks in the learning process, other irrelevant tasks are equivalent to noise, and adding noise is It can improve the generalization ability of the model. When performing different classification tasks, if multiple classification tasks are regarded as independent problems, each task must train a model to obtain results, and then add these results together, which is not only computationally time-consuming, but also Split the relationship between the various tasks. Multi-task learning shares the underlying hidden layer parameters across all tasks while reserving a few task-specific output layers to achieve multi-task. Multi-task learning involves parallel learning of multiple related tasks at the same time. The sharing of underlying parameters can help learning improve the generalizati...
Embodiment
[0069] The present invention has carried out accuracy comparison and analysis experiments on the above-mentioned improved model and the MT-DNN model on the open Chinese data set than the original MT-DNN network, specifically as follows:
[0070] The CrossWOZ data set is a Chinese large-scale multi-domain task-oriented dialogue data set, including three parts: training set, verification set and test set. The data set contains 6k dialogues and 102K sentences, involving 5 fields. The training set consists of 5012 dialogues, the validation set consists of 500 dialogues, and the test set has 500 dialogues. Each dialogue includes specific sentence text information, domain information, and intent-filled slot information, which can be used for multi-task natural language understanding.
[0071] In the experiment, the deep learning framework used is tensorflow. The new BERTCONTEXT model uses the word vectors trained by the BERT language model. In the improved BERTCONTEXT model, the BE...
PUM
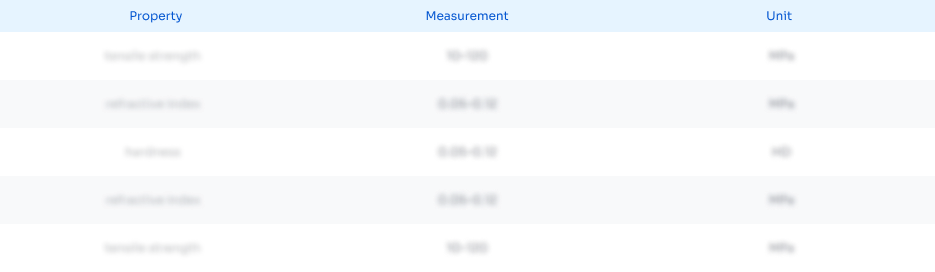
Abstract
Description
Claims
Application Information
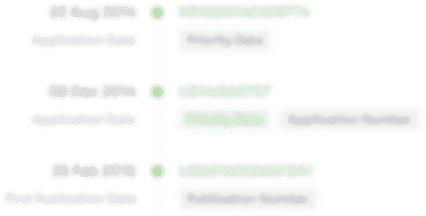
- R&D Engineer
- R&D Manager
- IP Professional
- Industry Leading Data Capabilities
- Powerful AI technology
- Patent DNA Extraction
Browse by: Latest US Patents, China's latest patents, Technical Efficacy Thesaurus, Application Domain, Technology Topic, Popular Technical Reports.
© 2024 PatSnap. All rights reserved.Legal|Privacy policy|Modern Slavery Act Transparency Statement|Sitemap|About US| Contact US: help@patsnap.com