A text classification method and device
A text classification and text technology, applied in the field of artificial intelligence, can solve problems such as low classification accuracy, unfavorable text processing, and failure to meet classification requirements, and achieve high efficiency and high classification accuracy
- Summary
- Abstract
- Description
- Claims
- Application Information
AI Technical Summary
Problems solved by technology
Method used
Image
Examples
Embodiment 1
[0037] see figure 1 As shown, it is a flow chart of the text classification method provided in Embodiment 1 of the present application. The method includes steps S101 to S104, wherein:
[0038] S101: Obtain the text to be classified, and determine the number of times each sample word in the sample vocabulary set appears in the text to be classified; the sample words in the sample vocabulary set are sample words used for text classification based on the text classification sub-model.
[0039] S102: Divide the sample vocabulary into multiple groups according to the sample vocabulary respectively used by the multiple text classification sub-models; wherein, each group corresponds to a text classification sub-model, and the sample vocabulary in different groups is not completely the same.
[0040] S103: Input the number of occurrences of the sample words in each group in the text to be classified into the text classification sub-model corresponding to each group, and obtain the sub-...
Embodiment 2
[0161] The embodiment of this application provides a method for processing problematic work orders, including:
[0162] (1) Collect 4,100 problem tickets generated in 2017 and manually marked with the actual classification results. The text content corresponding to the problem ticket includes: title, brief description, solution, etc.
[0163] There are 42 corresponding actual classification results, including: "resource management", "dual machine hot standby", "operating system and database", "installation, deployment and upgrade", "DBMAN", "alarm management", "topology management "Wait.
[0164] (2) Merge the text content in each problem work order into a character string, and perform word segmentation processing on the synthesized character string, and obtain a total of 4601 sample words, which are: a 1 ,a 2 ,...,a 4601
[0165] (3) Calculate the importance score of each sample vocabulary: build a random forest model.
[0166] The importance score calculation process o...
Embodiment 3
[0180] refer to Figure 8 As shown, it is a schematic diagram of a text classification device provided in Embodiment 3 of the present application, and the device includes: an acquisition module 81, a grouping module 82, and a classification module 83; wherein:
[0181] The obtaining module 81 is used to obtain the text to be classified, and determine the number of times each sample vocabulary in the sample vocabulary set appears in the text to be classified; the vocabulary in the sample vocabulary set is the sample used for text classification based on the text classification sub-model vocabulary;
[0182] The grouping module 82 is used to divide the sample vocabulary into multiple groups according to the sample vocabulary used by multiple text classification sub-models; wherein, each group corresponds to a text classification sub-model, and the sample vocabulary in different groups is not completely the same ;
[0183] The classification module 83 is used to input the number ...
PUM
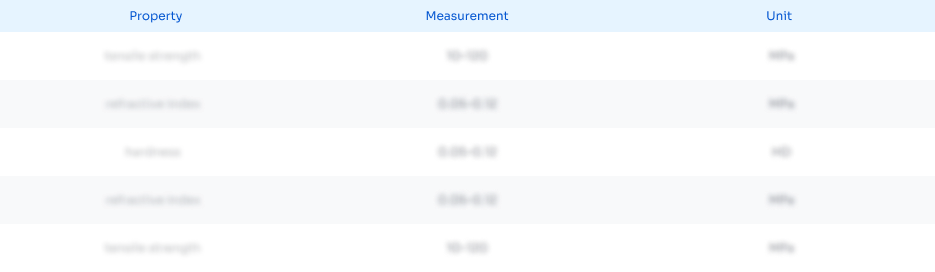
Abstract
Description
Claims
Application Information
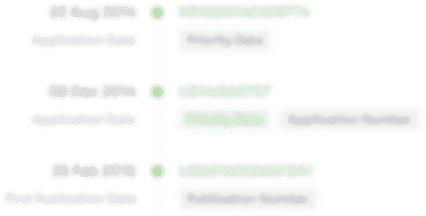
- R&D
- Intellectual Property
- Life Sciences
- Materials
- Tech Scout
- Unparalleled Data Quality
- Higher Quality Content
- 60% Fewer Hallucinations
Browse by: Latest US Patents, China's latest patents, Technical Efficacy Thesaurus, Application Domain, Technology Topic, Popular Technical Reports.
© 2025 PatSnap. All rights reserved.Legal|Privacy policy|Modern Slavery Act Transparency Statement|Sitemap|About US| Contact US: help@patsnap.com