Lithium ion battery remaining life prediction method based on wolf pack optimization LSTM network
A lithium-ion battery life prediction technology, applied in the field of lithium-ion batteries, can solve problems such as poor adaptability and inability to adapt to prediction problems
- Summary
- Abstract
- Description
- Claims
- Application Information
AI Technical Summary
Problems solved by technology
Method used
Image
Examples
Embodiment Construction
[0065] The specific implementation manners of the present invention will be further described in detail below in conjunction with the accompanying drawings and embodiments. The following examples are used to illustrate the present invention, but are not intended to limit the scope of the present invention.
[0066] In this embodiment, the lithium-ion battery degradation data from NASA Prognostic Center of Excellence (PCoE) is used, and the first group of lithium-ion battery sample battery capacity data labeled B0005 is selected as a specific implementation case data used in . The remaining life prediction method of the lithium ion battery based on the gray wolf group optimization LSTM network of the present invention is used to predict the remaining life of the lithium ion battery.
[0067] Lithium-ion battery remaining life prediction method based on gray wolf pack optimized LSTM network, such as figure 1 shown, including the following steps:
[0068] Step 1. Obtain the mo...
PUM
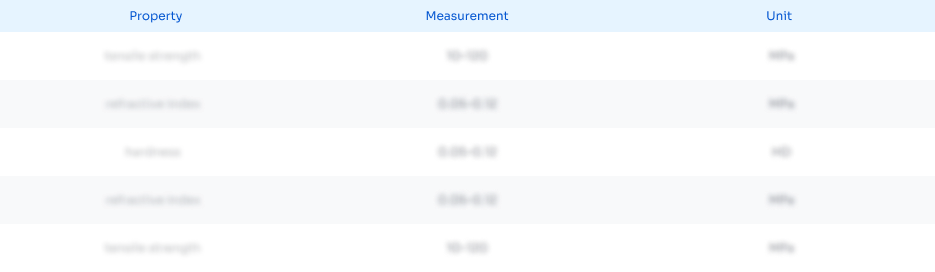
Abstract
Description
Claims
Application Information
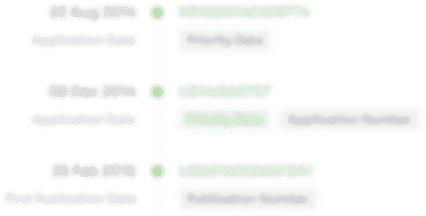
- R&D Engineer
- R&D Manager
- IP Professional
- Industry Leading Data Capabilities
- Powerful AI technology
- Patent DNA Extraction
Browse by: Latest US Patents, China's latest patents, Technical Efficacy Thesaurus, Application Domain, Technology Topic.
© 2024 PatSnap. All rights reserved.Legal|Privacy policy|Modern Slavery Act Transparency Statement|Sitemap