Image significance detection method based on confrontation network
An inspection method, a significant technology, applied in image enhancement, image analysis, image data processing, etc., to achieve the effect of improving accuracy
- Summary
- Abstract
- Description
- Claims
- Application Information
AI Technical Summary
Problems solved by technology
Method used
Image
Examples
Embodiment Construction
[0027] The invention uses confrontation training to realize the regularization function of the convolutional neural network, thereby improving the accuracy rate when using the neural network to predict. Aiming at the specific problem—significance prediction, the present invention proposes a data-driven regression method. The learning process can be described as a cost function that minimizes the Euclidean distance between the saliency map and the ground truth. In order to avoid getting local minimum values, the present invention uses a smaller batch size -2, although the convergence is slower, the effect is better. The combination of stochastic gradient descent method and impulse unit is used in the training process, which helps to escape the local minimum during the training process, so that the network can converge more quickly. The learning rate is gradually reduced to ensure that the optimal solution is not skipped when the step is too large in the process of finding the ...
PUM
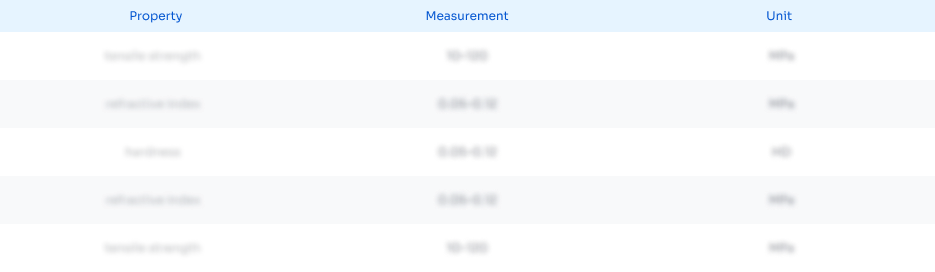
Abstract
Description
Claims
Application Information
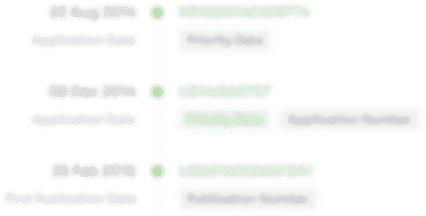
- R&D
- Intellectual Property
- Life Sciences
- Materials
- Tech Scout
- Unparalleled Data Quality
- Higher Quality Content
- 60% Fewer Hallucinations
Browse by: Latest US Patents, China's latest patents, Technical Efficacy Thesaurus, Application Domain, Technology Topic, Popular Technical Reports.
© 2025 PatSnap. All rights reserved.Legal|Privacy policy|Modern Slavery Act Transparency Statement|Sitemap|About US| Contact US: help@patsnap.com