Image segmentation method based on multi-supervision full-convolution neural network
A convolutional neural network and image segmentation technology, applied in the field of medical image processing, can solve the problems of poor segmentation efficiency, large amount of calculation, and insufficient feature extraction, and achieve the effect of reducing segmentation errors, speeding up training speed, and improving segmentation accuracy.
- Summary
- Abstract
- Description
- Claims
- Application Information
AI Technical Summary
Problems solved by technology
Method used
Image
Examples
Embodiment Construction
[0025] Such as figure 1 Shown, the segmentation method of a kind of multi-supervision fully convolutional neural network of the present invention, specific technical details are as follows:
[0026] (1) Collect CT images of osteosarcoma and preprocess the images;
[0027] First, anisotropic diffusion filter algorithm is used to denoise the input image, and then the denoised image is standardized to obtain the normalized image.
[0028] (2) Train a multi-supervised fully convolutional neural network model.
[0029] The images normalized in the first step and the labeled images are input into a multi-supervised fully convolutional neural network for training.
[0030] 1) Multi-supervised fully convolutional network structure.
[0031] Such as figure 2 As shown, the multi-supervised fully convolutional neural network consists of two parts: the convolution part and the supervised edge output layer network part. The original network structure of the vgg-16 model before conv5_3 ...
PUM
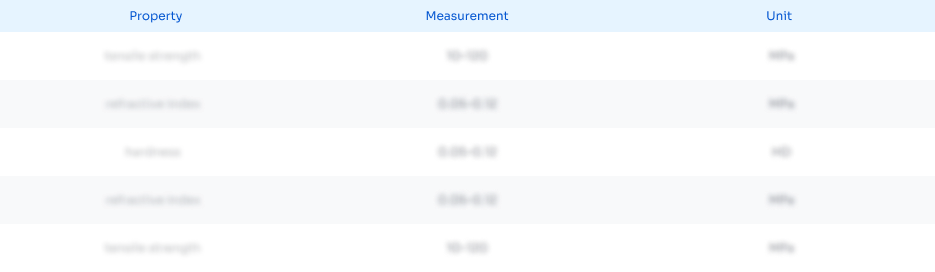
Abstract
Description
Claims
Application Information
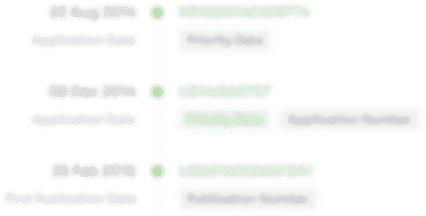
- R&D Engineer
- R&D Manager
- IP Professional
- Industry Leading Data Capabilities
- Powerful AI technology
- Patent DNA Extraction
Browse by: Latest US Patents, China's latest patents, Technical Efficacy Thesaurus, Application Domain, Technology Topic, Popular Technical Reports.
© 2024 PatSnap. All rights reserved.Legal|Privacy policy|Modern Slavery Act Transparency Statement|Sitemap|About US| Contact US: help@patsnap.com