Clustering analysis method and system under non-independent identical distribution
A cluster analysis, non-independent technology, applied in the direction of instruments, character and pattern recognition, computer components, etc., can solve the problem that category data cannot effectively obtain attribute values, and achieve the effect of avoiding human subjectivity and improving quality
- Summary
- Abstract
- Description
- Claims
- Application Information
AI Technical Summary
Problems solved by technology
Method used
Image
Examples
Embodiment 1
[0041] This embodiment provides a new non-independent and identically distributed clustering analysis method using coupling similarity for measurement. This method solves the problem of how to capture the real relationship in the category data set, and obtains it based on the NI-DBSCANS clustering algorithm. More accurate and efficient clustering results can accurately calculate the real relationship between attribute values of category data, thereby improving the quality of clustering results.
[0042] Please refer to the attached figure 1 , the non-independent and identically distributed cluster analysis method includes the following steps:
[0043] S101. Obtain a data set and create an information table S.
[0044] Specifically, in the step 101, the data set U is obtained, and the data set U is preprocessed, and the information table S is formed by using the preprocessed data set. The information table S is shown in Table 1, and the row in the information table S Repres...
Embodiment 2
[0102] In order to enable those skilled in the art to better understand the technical solution of the present application, a more detailed embodiment is listed below. This embodiment provides a non-independent and identically distributed cluster analysis method for mining and analyzing data , this embodiment has the following settings:
[0103] The formula for calculating the accuracy of clustering results is:
[0104]
[0105] Among them, n represents the correct clustering result, and N represents the total number of objects in the dataset.
[0106] Please refer to the attached figure 2 , the non-independent and identically distributed cluster analysis method includes the following steps:
[0107] S201, acquire the Zoo data set, and establish the information table S 1 .
[0108] Specifically, the Zoo data set has a total of 101 objects and 16 attributes (hair, feathers, eggs, milk, flying, aquatic, predation, teeth, backbone, breath, poison, fins, legs, tail, domesti...
Embodiment 3
[0154] This embodiment provides a cluster analysis system under non-independent and identical distribution, please refer to the attached Figure 5 , the system consists of:
[0155] The coupling similarity matrix generation module is used to obtain the data set and establish the information table; calculate the coupling similarity between the data objects in the information table to obtain the coupling similarity matrix;
[0156] The coupling similarity matrix analysis module analyzes the coupling similarity matrix, and calculates the Eps neighborhood interval value and the core point threshold Minpts;
[0157] The clustering module performs clustering analysis on the information table based on the obtained Eps neighborhood interval value and the core point threshold value Minpts.
PUM
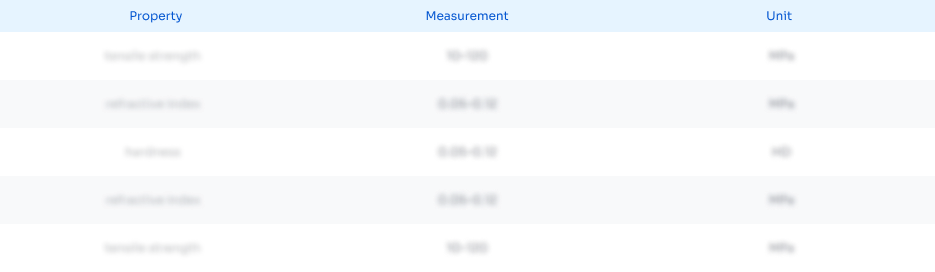
Abstract
Description
Claims
Application Information
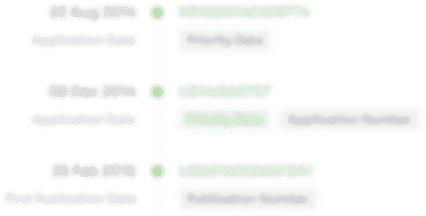
- R&D Engineer
- R&D Manager
- IP Professional
- Industry Leading Data Capabilities
- Powerful AI technology
- Patent DNA Extraction
Browse by: Latest US Patents, China's latest patents, Technical Efficacy Thesaurus, Application Domain, Technology Topic, Popular Technical Reports.
© 2024 PatSnap. All rights reserved.Legal|Privacy policy|Modern Slavery Act Transparency Statement|Sitemap|About US| Contact US: help@patsnap.com