An Incremental Spatiotemporal Learning Method for Online Modeling of Distributed Parameter Systems
A learning method and distributed technology, applied in general control systems, control/regulation systems, instruments, etc., can solve the problems of online updating of space-time synthetic sets, data identification of space-time synthetic sets, time-consuming problems, etc., and achieve remarkable calculation results , broad application prospects, the effect of reducing time and memory usage
- Summary
- Abstract
- Description
- Claims
- Application Information
AI Technical Summary
Problems solved by technology
Method used
Image
Examples
Embodiment 1
[0143] This example presents an incremental spatiotemporal learning method for online modeling of distributed parameter systems, including:
[0144] (1) After adding new data to the incremental data set, incrementally update the spatial basis functions;
[0145] (2) Update the time coefficient and identify the new timing model;
[0146] (3) Reconstruct historical data through the old space-time composite set, the updated spatial basis function in step (1) and the time series model identified in step (2), and predict future output;
[0147] (4) Repeat steps (1)-(3) to complete the online update of the spatio-temporal synthetic set.
[0148] Among them, the data increment set is the collected continuous data flow with a specific time step, the spatial basis function is the basis function of n-dimensional space, and the basis function of n-dimensional space is separated by time and space, with the time step as L, learned from the training data. , Incremental update refers to t...
Embodiment 2
[0156] In order to prove the performance of the incremental space-time learning method for online modeling of distributed parameter systems in Example 1, we take the catalytic reaction rod experiment as an example, and compare Example 1 with the traditional batch processing mode method to illustrate Feasibility and advantages of incremental learning.
PUM
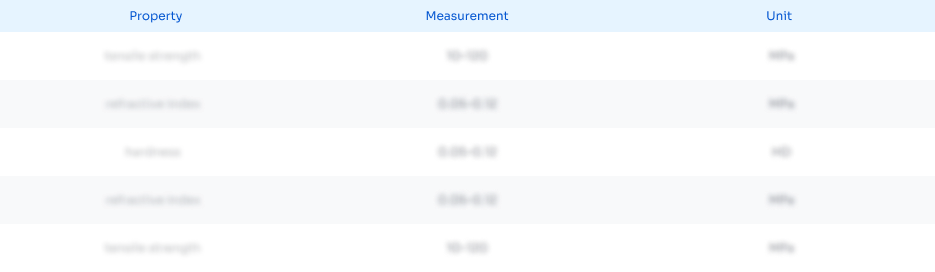
Abstract
Description
Claims
Application Information
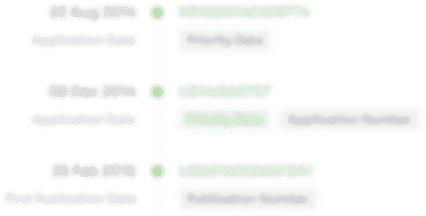
- R&D
- Intellectual Property
- Life Sciences
- Materials
- Tech Scout
- Unparalleled Data Quality
- Higher Quality Content
- 60% Fewer Hallucinations
Browse by: Latest US Patents, China's latest patents, Technical Efficacy Thesaurus, Application Domain, Technology Topic, Popular Technical Reports.
© 2025 PatSnap. All rights reserved.Legal|Privacy policy|Modern Slavery Act Transparency Statement|Sitemap|About US| Contact US: help@patsnap.com