Deep Hash pedestrian re-identification method
A technology of pedestrian re-identification and deep hashing, which is applied in the field of pedestrian re-identification, can solve the problems of Hamming distance loss that is not easy to converge, and achieve the effect of benefiting loss calculation and model convergence, improving accuracy and reducing time
- Summary
- Abstract
- Description
- Claims
- Application Information
AI Technical Summary
Problems solved by technology
Method used
Image
Examples
Embodiment
[0104] 1. Dataset
[0105] Using the Market1501 dataset, collected by Zheng et al. in the campus scene and released in 2015, the dataset contains 1501 pedestrian ids, taken by 6 cameras, with a total of 32217 images.
[0106] 2. Experimental settings
[0107] The training set has 1501 pedestrian ids. In the process of testing and training, 751 pedestrian pictures with ids are selected as the training set, and the pictures with the remaining 750 ids are used as the test set; in the experiment, set λ th = 1, λ qt = 0.001, λ cons =0.01, β=1, learning rate 3*10 -4 , the learning rate decreases exponentially after reaching 150epoch.
[0108] 3. Training and testing methods
[0109] Training phase: Send pictures to the network in batches for training, set the batchsize to 128, and generate gradient backpropagation update (SGD) under loss supervision. After 300 Epoch iterations, the final network model is obtained.
PUM
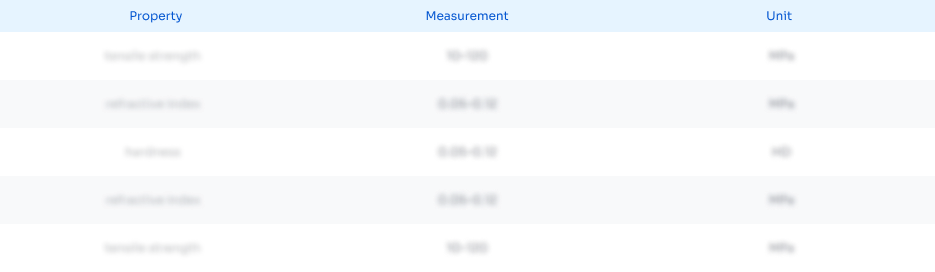
Abstract
Description
Claims
Application Information
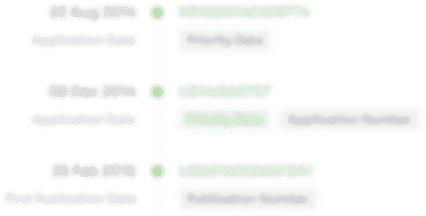
- R&D Engineer
- R&D Manager
- IP Professional
- Industry Leading Data Capabilities
- Powerful AI technology
- Patent DNA Extraction
Browse by: Latest US Patents, China's latest patents, Technical Efficacy Thesaurus, Application Domain, Technology Topic, Popular Technical Reports.
© 2024 PatSnap. All rights reserved.Legal|Privacy policy|Modern Slavery Act Transparency Statement|Sitemap|About US| Contact US: help@patsnap.com