RNA secondary structure prediction method based on recurrent neural network
A recursive neural network and secondary structure technology, applied in the field of biological research, can solve the problems of increased prediction time and cost, difficult to obtain crystals, and long time-consuming secondary structure, so as to improve the prediction accuracy.
- Summary
- Abstract
- Description
- Claims
- Application Information
AI Technical Summary
Problems solved by technology
Method used
Image
Examples
Embodiment 1
[0035] Embodiment 1: RNA secondary structure prediction method based on recursive neural network, carry out according to the following steps: download and obtain PDB data set from PDB database official website, such as figure 1Shown is a partial example of one of the RNA information '2JTP.pdb' in the downloaded PDB data. The PDB database contains three parts: RNA sequence information, RNA primary sequence and three-dimensional space coordinates. It can be seen from the figure The primary sequence of RNA is recorded in 'SEQRES'. Firstly, data preprocessing is performed on the PDB data set, and the primary sequence is extracted by means of regular expressions. Some of the data are except A, C, G, U For other characters, these characters need to be cleaned to obtain the correct RNA primary sequence. The modeling method of the machine learning model selects the SVM package in the scikit-learn package in the python extension library for direct modeling, and selects the Gaussian ker...
PUM
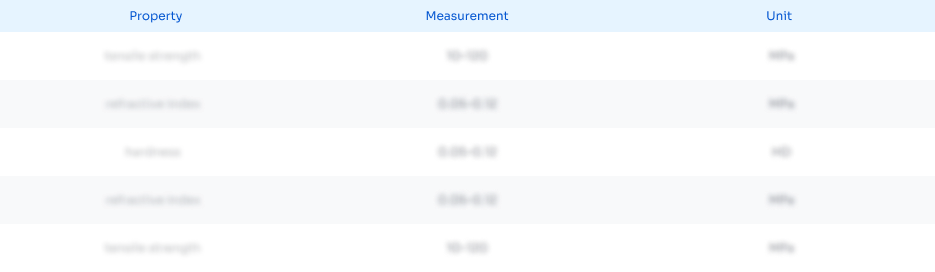
Abstract
Description
Claims
Application Information
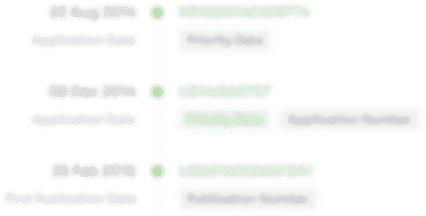
- R&D Engineer
- R&D Manager
- IP Professional
- Industry Leading Data Capabilities
- Powerful AI technology
- Patent DNA Extraction
Browse by: Latest US Patents, China's latest patents, Technical Efficacy Thesaurus, Application Domain, Technology Topic, Popular Technical Reports.
© 2024 PatSnap. All rights reserved.Legal|Privacy policy|Modern Slavery Act Transparency Statement|Sitemap|About US| Contact US: help@patsnap.com