3D printing model defect pre-judgment system and method based on visual neural learning
A 3D printing and visual nerve technology, applied in image data processing, instruments, computing, etc., can solve problems such as poor printing materials and processing accuracy, long production time of 3D printing models, inaccurate control of 3D printing conditions, etc., to achieve Great practical value, enhance user experience, and improve printing efficiency
- Summary
- Abstract
- Description
- Claims
- Application Information
AI Technical Summary
Problems solved by technology
Method used
Image
Examples
Embodiment 1
[0036] Such as figure 1 , 2As shown, a 3D printing model defect prediction system based on visual neural learning includes a scanning unit, an information transmission unit and an information processing module; wherein, the scanning unit includes a camera 2 and a grating projection device 3, and the information transmission unit includes a The WiFi module 5 and the controller 4, the grating projection device 3 projects the grating on the object to be measured, and changes the thickness and displacement, cooperates with the camera 2 to transmit the captured digital image to the controller 4, and the controller 4 passes the WiFi module 5 is transmitted to the information processing module 1, and the information processing module 1 uploads the obtained digital processing information to the system server, and at the same time, the information processing module inputs the frame-by-frame image data into the system trained convolutional neural network model library for matching and p...
Embodiment 2
[0044] Such as image 3 As shown, a 3D printing model defect prediction method based on visual neural learning includes the following steps:
[0045] S1: using the grating projection device 3 to perform grating projection on the object to be measured;
[0046] S2: use the camera 2 to take pictures of the object to be tested;
[0047] S3: transmit the digital image of the object under test acquired by the grating projection device 3 and the camera 2 to the controller 4, and the controller 4 transmits it to the information processing module 1 through the WiFi module 5;
[0048] S4: The information processing module 1 uploads the obtained digital processing information to the system server, and at the same time, the information processing module 1 inputs the frame-by-frame image data into the convolutional neural network model trained by the system for matching and prediction;
[0049] S5: The automatic defect prediction unit predicts whether the model printed by the user is a ...
PUM
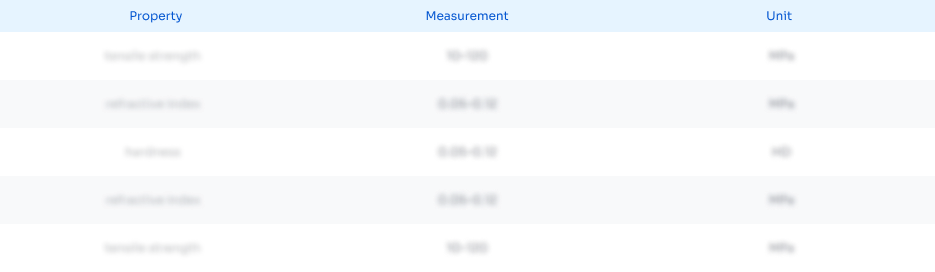
Abstract
Description
Claims
Application Information
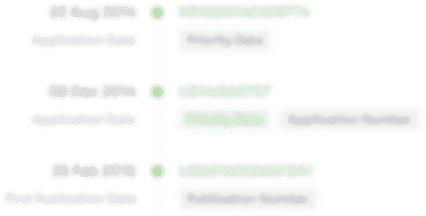
- R&D Engineer
- R&D Manager
- IP Professional
- Industry Leading Data Capabilities
- Powerful AI technology
- Patent DNA Extraction
Browse by: Latest US Patents, China's latest patents, Technical Efficacy Thesaurus, Application Domain, Technology Topic.
© 2024 PatSnap. All rights reserved.Legal|Privacy policy|Modern Slavery Act Transparency Statement|Sitemap