Image fine-grained recognition method based on multi-scale feature fusion
A multi-scale feature and recognition method technology, applied in character and pattern recognition, instruments, biological neural network models, etc., can solve problems such as poor real-time requirements
- Summary
- Abstract
- Description
- Claims
- Application Information
AI Technical Summary
Problems solved by technology
Method used
Image
Examples
Embodiment Construction
[0035] Below in conjunction with accompanying drawing and specific embodiment the present invention is described in further detail:
[0036] The present invention provides an image fine-grained recognition method based on multi-scale feature fusion. The present invention uses a feature pyramid method to fuse multi-layer bilinear features, independently predicts fine-grained recognition results at each layer, and finally votes the prediction results of each layer. , to get the final fine-grained recognition result. The present invention is tested on the cigarette fine-grained recognition data set Cigarette67-2018 and the public bird fine-grained recognition data set CUB200-2011 proposed by the laboratory. With improvement, the accuracies on the above two test sets are 85.4% and 95.95%, respectively. On the other hand, the real-time reasoning speed of the present invention on a single-core CPU can meet the real-time requirement.
[0037] Taking the public data set CUB200-2011 ...
PUM
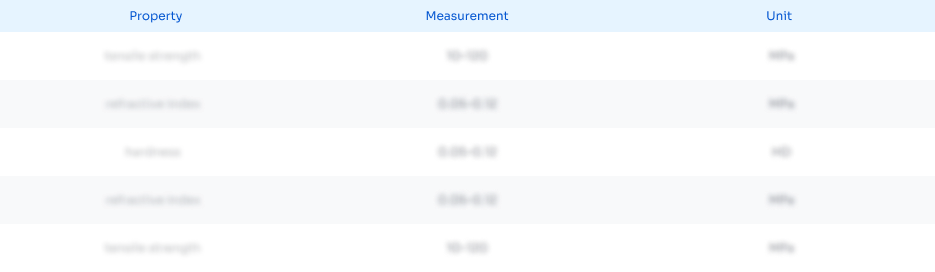
Abstract
Description
Claims
Application Information
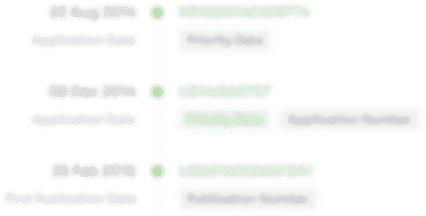
- R&D Engineer
- R&D Manager
- IP Professional
- Industry Leading Data Capabilities
- Powerful AI technology
- Patent DNA Extraction
Browse by: Latest US Patents, China's latest patents, Technical Efficacy Thesaurus, Application Domain, Technology Topic, Popular Technical Reports.
© 2024 PatSnap. All rights reserved.Legal|Privacy policy|Modern Slavery Act Transparency Statement|Sitemap|About US| Contact US: help@patsnap.com