Gas compressor characteristic prediction method based on Kriging model optimization and neural network
A kriging model and neural network technology, applied in the field of compressor characteristic prediction, to achieve high calculation accuracy
- Summary
- Abstract
- Description
- Claims
- Application Information
AI Technical Summary
Problems solved by technology
Method used
Image
Examples
Embodiment Construction
[0048] The present invention is described in more detail below in conjunction with accompanying drawing example:
[0049] combine figure 1 , choose two kriging methods, namely the univariate ordinary kriging method and the univariate universal kriging method. According to the drift of the data source, different kriging methods need to be selected. For each Kriging method, 3 semivariograms are used to solve the Kriging equations, 6 sets of weighting coefficients are obtained, and 6 compressor characteristic prediction surfaces are obtained by fitting.
[0050] Using the improved leave-one-out cross-validation method, the original sample data is divided into a training sample set and a test sample set, the model is first trained on the training sample set, and then the prediction error is obtained on the test sample set. The improved leave-one-out cross-validation method means that in order to ensure that each prediction is an interpolation prediction, the data on the speed lin...
PUM
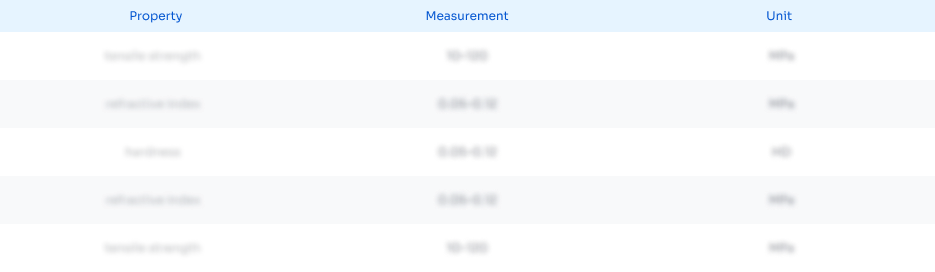
Abstract
Description
Claims
Application Information
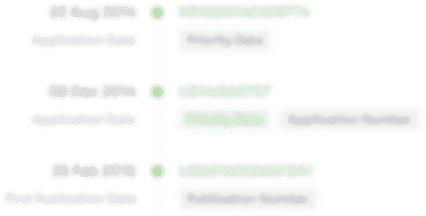
- R&D
- Intellectual Property
- Life Sciences
- Materials
- Tech Scout
- Unparalleled Data Quality
- Higher Quality Content
- 60% Fewer Hallucinations
Browse by: Latest US Patents, China's latest patents, Technical Efficacy Thesaurus, Application Domain, Technology Topic, Popular Technical Reports.
© 2025 PatSnap. All rights reserved.Legal|Privacy policy|Modern Slavery Act Transparency Statement|Sitemap|About US| Contact US: help@patsnap.com