Depth correlation target tracking algorithm based on mutual reinforcement and multi-attention mechanism learning
A target tracking and attention mechanism technology, applied in the field of image processing, can solve problems such as information redundancy, insufficiency, single low-level feature expression, etc., to achieve the effects of improving robustness, improving effective distribution, and alleviating boundary effects
- Summary
- Abstract
- Description
- Claims
- Application Information
AI Technical Summary
Problems solved by technology
Method used
Image
Examples
Embodiment
[0034] The depth correlation tracking algorithm of mutual reinforcement and multi-attention mechanism learning provided in this embodiment, the process is as follows figure 1 , figure 2 , image 3 , Figure 4 , Figure 5 Shown, specifically include the following steps:
[0035] S1: Input the previous frame to get the target area;
[0036] S2: Establish a feature extractor that is beneficial to tracking, and extract the target area features of S1 through the feature extractor, such as image 3 , the feature extractor is composed of a convolutional network, an encoder and a decoder. It is improved on the original tracking algorithm DCFNet. The original DCFNet algorithm only contains shallow features obtained by two convolutions, and the encoder and decoder are added. The EDNet structure of the decoder extracts high-level semantic information, which combines shallow features and sends them to channel attention and spatial attention mechanisms after fusion, such as Figure ...
PUM
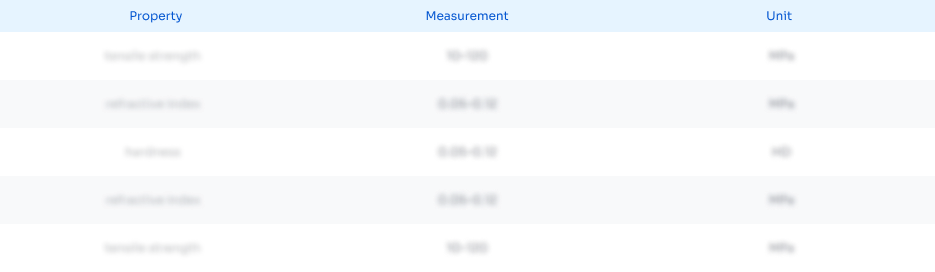
Abstract
Description
Claims
Application Information
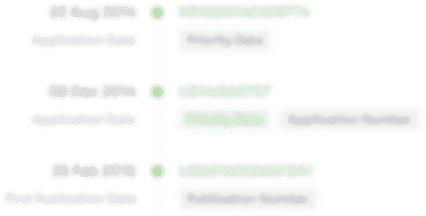
- R&D Engineer
- R&D Manager
- IP Professional
- Industry Leading Data Capabilities
- Powerful AI technology
- Patent DNA Extraction
Browse by: Latest US Patents, China's latest patents, Technical Efficacy Thesaurus, Application Domain, Technology Topic, Popular Technical Reports.
© 2024 PatSnap. All rights reserved.Legal|Privacy policy|Modern Slavery Act Transparency Statement|Sitemap|About US| Contact US: help@patsnap.com