Microgrid short-term load prediction method based on long-term and short-term memory and self-adaptive improvement
A technology of short-term load forecasting and long-term short-term memory, applied in forecasting, neural learning methods, data processing applications, etc., can solve the problems of low forecasting accuracy, achieve the effect of improving forecasting accuracy and overcoming high randomness and complexity
- Summary
- Abstract
- Description
- Claims
- Application Information
AI Technical Summary
Problems solved by technology
Method used
Image
Examples
Embodiment Construction
[0046] Embodiments of the invention are described in detail below, examples of which are illustrated in the accompanying drawings. The embodiments described below by referring to the figures are exemplary and are intended to explain the present invention and should not be construed as limiting the present invention.
[0047] Combine below Figure 1-Figure 8 The short-term load forecasting method of microgrid based on long-short-term memory and self-adaptive improvement is illustrated with an example.
[0048] see figure 1 and figure 2 As shown, a microgrid short-term load forecasting method based on long-term short-term memory and adaptive improvement, the forecasting method includes:
[0049] Step 1. Collect the basic data of the microgrid and retrieve the historical load data of the microgrid.
[0050] Generally, the basic data of the microgrid includes information such as the construction time, geographical location, equipment capacity and load type of the microgrid in...
PUM
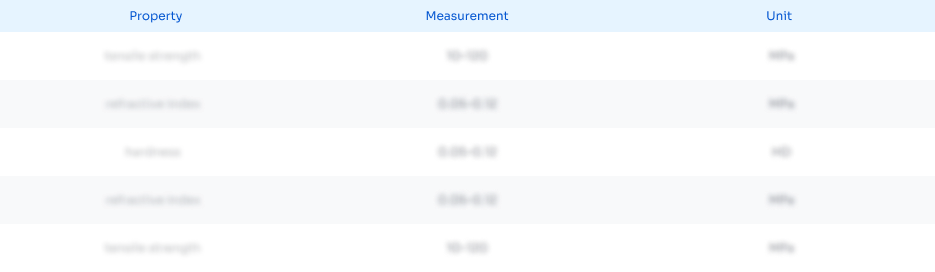
Abstract
Description
Claims
Application Information
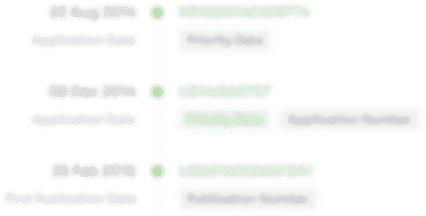
- R&D Engineer
- R&D Manager
- IP Professional
- Industry Leading Data Capabilities
- Powerful AI technology
- Patent DNA Extraction
Browse by: Latest US Patents, China's latest patents, Technical Efficacy Thesaurus, Application Domain, Technology Topic, Popular Technical Reports.
© 2024 PatSnap. All rights reserved.Legal|Privacy policy|Modern Slavery Act Transparency Statement|Sitemap|About US| Contact US: help@patsnap.com