Medical image classification method based on self-weighted grading biological characteristic, device and computer-readable storage medium
A biometric, medical image technology, applied in medical images, computer parts, computer-aided medical procedures, etc., can solve the problem that pMCI and sMCI cannot be effectively distinguished, cannot fully reflect the bias of MCI patients, and information cannot accurately transmit MCI. Patients and other problems, to achieve the effect of improving classification accuracy, strong discrimination and robustness
- Summary
- Abstract
- Description
- Claims
- Application Information
AI Technical Summary
Problems solved by technology
Method used
Image
Examples
Embodiment Construction
[0036]The present invention will be further described below in conjunction with accompanying drawing and example.
[0037] Such as figure 1 As shown, the specific steps of the medical image classification method based on self-weighting grading biological features of the present invention for pMCI and sMCI classification are as follows:
[0038] (1) Image preprocessing
[0039] The MRI images of 142 AD patients, 165 normal subjects, 126 pMCI patients and 95 sMCI patients were randomly selected from the ADNI database (such as figure 2 shown), using FreeSurfer software for preprocessing to extract two morphological features of cortical thickness (cortical thickness, CT) and volume (volume, VOL). CT refers to the closest distance between the white and gray matter surfaces in each vertex. Then, the image is smoothed using a Gaussian kernel function. Finally, the mean CT and VOL of each anatomical region were calculated using the Automated Anatomical Labeling (AAL) template. I...
PUM
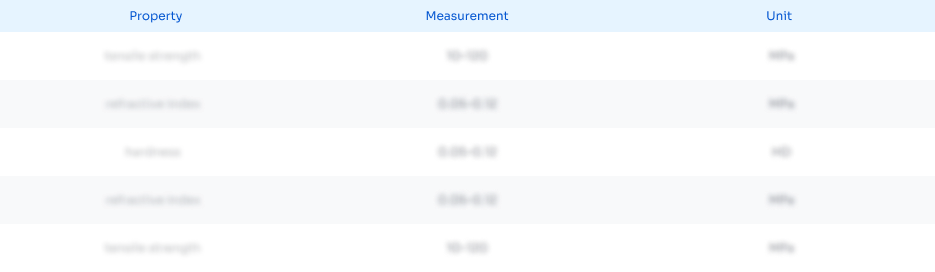
Abstract
Description
Claims
Application Information
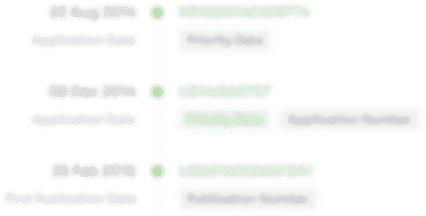
- R&D Engineer
- R&D Manager
- IP Professional
- Industry Leading Data Capabilities
- Powerful AI technology
- Patent DNA Extraction
Browse by: Latest US Patents, China's latest patents, Technical Efficacy Thesaurus, Application Domain, Technology Topic, Popular Technical Reports.
© 2024 PatSnap. All rights reserved.Legal|Privacy policy|Modern Slavery Act Transparency Statement|Sitemap|About US| Contact US: help@patsnap.com