Gas pipeline leak recognition method based on convolutional neural network
A convolutional neural network and gas pipeline technology, applied in biological neural network models, pipeline systems, neural architectures, etc., can solve the problems of time-consuming and labor-intensive false alarm rate and false alarm rate, and reduce the work of sound signal preprocessing. Effect
- Summary
- Abstract
- Description
- Claims
- Application Information
AI Technical Summary
Problems solved by technology
Method used
Image
Examples
Embodiment Construction
[0037] Embodiments of the present invention are described in detail below, and the embodiments are exemplary and intended to explain the present invention, but should not be construed as limiting the present invention.
[0038] Such as figure 1 As shown, the convolutional neural network-based gas pipeline leakage identification method in this embodiment includes the following steps:
[0039] Step 1: Through valve opening, gasket drilling, and pipe wall drilling, three typical leakage types, which are most likely to occur in actual gas pipelines, are simulated: loose valve leakage, gasket aging leakage, and pipe wall corrosion and damage leakage. Microphone arrays are used to collect three types of leakage acoustic signals and background acoustic signals that simulate typical leakage types; and multiple acquisitions are made by adjusting the size of the valve opening, replacing gaskets and pipe walls with different apertures, so as to obtain as many different leaks as possible....
PUM
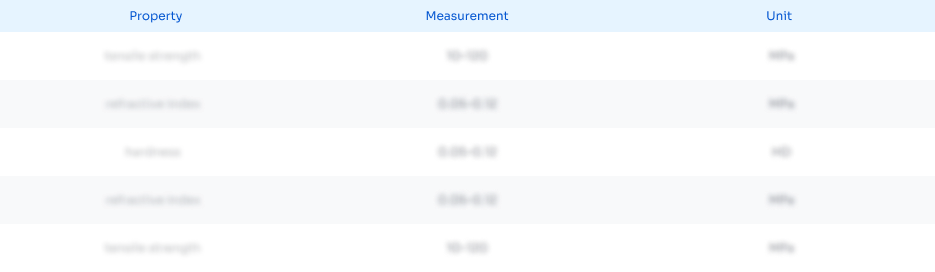
Abstract
Description
Claims
Application Information
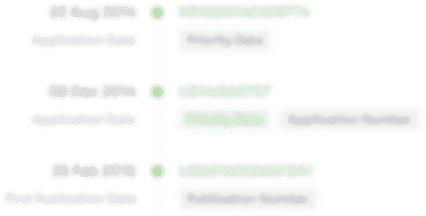
- R&D Engineer
- R&D Manager
- IP Professional
- Industry Leading Data Capabilities
- Powerful AI technology
- Patent DNA Extraction
Browse by: Latest US Patents, China's latest patents, Technical Efficacy Thesaurus, Application Domain, Technology Topic, Popular Technical Reports.
© 2024 PatSnap. All rights reserved.Legal|Privacy policy|Modern Slavery Act Transparency Statement|Sitemap|About US| Contact US: help@patsnap.com