Super-resolution reconstruction method based on dirac residual deep neural network
A deep neural network and super-resolution reconstruction technology, applied in the field of image reconstruction, can solve the problems of poor reconstruction effect and achieve good reconstruction effect
- Summary
- Abstract
- Description
- Claims
- Application Information
AI Technical Summary
Problems solved by technology
Method used
Image
Examples
Embodiment
[0043] (1) Data set preparation. The training set uses the training set part of the public dataset DIV2K. The test set includes the test set parts of Set5, Set14, B100, Urban100 and DIV2K. For each HR image, there are corresponding LR images (×2,×3,×4) as input.
[0044] (2) The network uses an image with a size of 48×48×3 as the input of the network. These images are obtained by cropping the dataset images. Since there are more than one input images for each iteration of training, n is used to represent the number of inputs, and the input is expressed as a tensor [n,48,48,3], and the corresponding output tensor is: ×2:[n,96, 96,3], ×3: [n,144,144,3], ×4: [n,192,192,3].
[0045] (3) The input image format is a 24-bit RGB image, and each pixel contains three-dimensional information of R, G, and B. Each dimension is represented by 0 to 255. Preprocessing is performed after the image is input, and each dimension of the image is represented by -127 to 128. The specific oper...
PUM
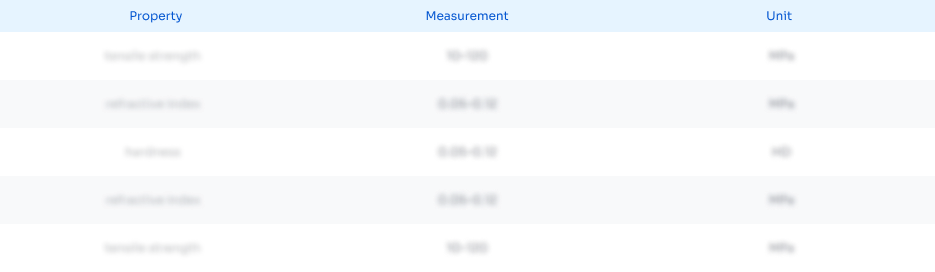
Abstract
Description
Claims
Application Information
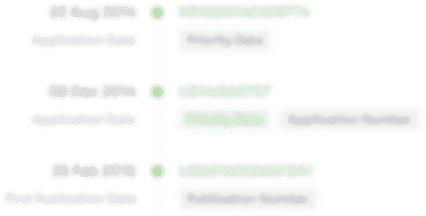
- R&D Engineer
- R&D Manager
- IP Professional
- Industry Leading Data Capabilities
- Powerful AI technology
- Patent DNA Extraction
Browse by: Latest US Patents, China's latest patents, Technical Efficacy Thesaurus, Application Domain, Technology Topic, Popular Technical Reports.
© 2024 PatSnap. All rights reserved.Legal|Privacy policy|Modern Slavery Act Transparency Statement|Sitemap|About US| Contact US: help@patsnap.com