Class identification method for zero sample picture
A sample picture and recognition method technology, applied in the field of picture recognition, can solve problems such as expensive labeling of attributes, difficulty in learning implicit relationship between categories, inapplicability of large-scale zero-sample picture category recognition, etc., to achieve the effect of improving accuracy
- Summary
- Abstract
- Description
- Claims
- Application Information
AI Technical Summary
Problems solved by technology
Method used
Image
Examples
example
[0085] The Hit@K metric is used to evaluate our model. Hit@k refers to the proportion of images with correct labels in the first k results returned by the model, and is the most commonly used classification evaluation method. In this example, k are 1, 2, 5, 10, 20, respectively.
[0086] The present invention is tested on the large-scale picture data set ImageNet 2011 21K data set. The data set contains 21841 categories, 1000 categories are selected as the training set (ImageNet 2012 1K), and the remaining 20841 categories are the test set. Divide the test set into three subsets, two hops (2-hops), three hops (3-hops) and all (All). Two hops means that the test set data is at most two nodes away from the training set, and three hops means that the test set data is at most three nodes away from the training set, all of which include all categories (20841 categories) in the ImageNet 2011 21K dataset. During the test, there are two settings to test respectively. The first is t...
PUM
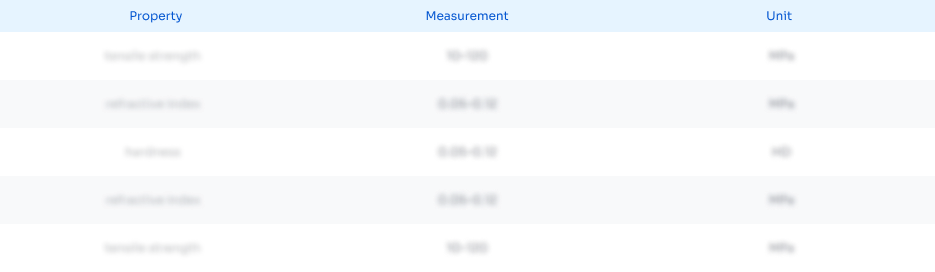
Abstract
Description
Claims
Application Information
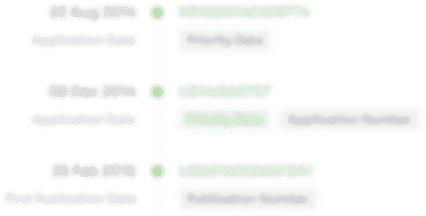
- R&D Engineer
- R&D Manager
- IP Professional
- Industry Leading Data Capabilities
- Powerful AI technology
- Patent DNA Extraction
Browse by: Latest US Patents, China's latest patents, Technical Efficacy Thesaurus, Application Domain, Technology Topic, Popular Technical Reports.
© 2024 PatSnap. All rights reserved.Legal|Privacy policy|Modern Slavery Act Transparency Statement|Sitemap|About US| Contact US: help@patsnap.com