Group distribution detection method based on Chebyshev filter
A Chebyshev and distribution detection technology, applied in the field of deep learning and complex network community discovery, can solve the problem of low computing efficiency, achieve the effect of improving efficiency and realizing network weight sharing
- Summary
- Abstract
- Description
- Claims
- Application Information
AI Technical Summary
Problems solved by technology
Method used
Image
Examples
Embodiment Construction
[0040] The group detection method based on the Chebyshev filter of the present invention will be further described below in conjunction with the accompanying drawings.
[0041] like figure 1 As shown, the present invention provides a group distribution detection method based on the Chebyshev filter.
[0042] For a complex social network formed by the college football league, the 115 college student teams participating in the competition were divided into 12 leagues, and 616 games were carried out. The specific process of group detection with the method of the present invention includes the following steps:
[0043] Step 1: Read complex network data, and abstract the complex network into a graph model, which includes nodes, edges, and adjacency weight matrix. The specific symbols are expressed as: G=(V, E, W), where V is The set of nodes represents the team, and E represents the set of nodes connecting edges, which means that two teams have played games. For each node v i ∈V,...
PUM
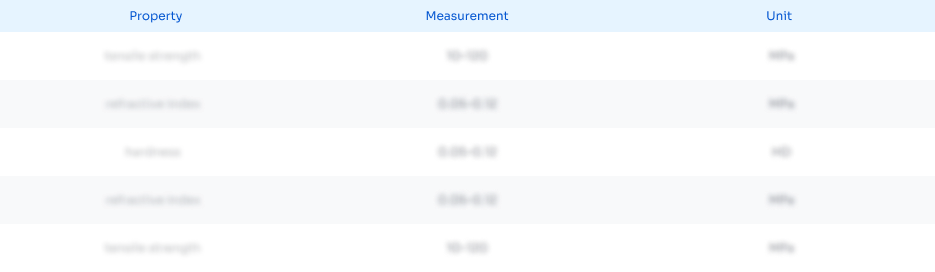
Abstract
Description
Claims
Application Information
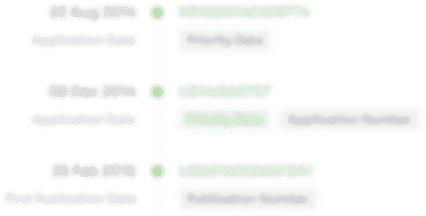
- R&D
- Intellectual Property
- Life Sciences
- Materials
- Tech Scout
- Unparalleled Data Quality
- Higher Quality Content
- 60% Fewer Hallucinations
Browse by: Latest US Patents, China's latest patents, Technical Efficacy Thesaurus, Application Domain, Technology Topic, Popular Technical Reports.
© 2025 PatSnap. All rights reserved.Legal|Privacy policy|Modern Slavery Act Transparency Statement|Sitemap|About US| Contact US: help@patsnap.com