Deep inverse reinforcement learning-based target detection method in unmanned aerial vehicle aerial video based on deep inverse reinforcement learning
A technology of reinforcement learning and target detection, which is used in computer parts, instruments, biological neural network models, etc.
- Summary
- Abstract
- Description
- Claims
- Application Information
AI Technical Summary
Problems solved by technology
Method used
Image
Examples
Embodiment Construction
[0083] A target detection method in the unmanned aerial vehicle aerial video of a kind of depth inverse reinforcement learning, it is characterized in that: comprise the following steps at least:
[0084] Step 1. Establish a deep inverse reinforcement learning model;
[0085] Step 2. Model strategy iteration and algorithm implementation;
[0086] Step 3. Selection and optimization of key parameters of the model.
[0087] Described step 1 comprises following specific steps:
[0088] Step 1, establish a deep inverse reinforcement learning model
[0089] Randomly select the video clips that have acquired the target during the training process as the model input. Under the premise of n-frame association detection, define the minimum number of frames of the video clip as n+1, and the maximum number of frames of the video clip does not exceed 2n, such as the formula (1 );
[0090] n+1≤FramCount≤2n (1)
[0091] Such as figure 1 As shown, the video clips in the obtained target a...
PUM
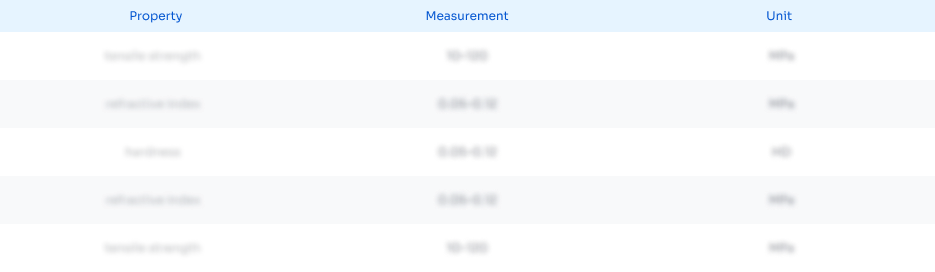
Abstract
Description
Claims
Application Information
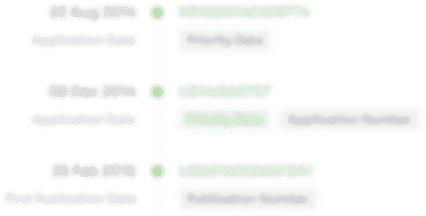
- R&D
- Intellectual Property
- Life Sciences
- Materials
- Tech Scout
- Unparalleled Data Quality
- Higher Quality Content
- 60% Fewer Hallucinations
Browse by: Latest US Patents, China's latest patents, Technical Efficacy Thesaurus, Application Domain, Technology Topic, Popular Technical Reports.
© 2025 PatSnap. All rights reserved.Legal|Privacy policy|Modern Slavery Act Transparency Statement|Sitemap|About US| Contact US: help@patsnap.com