Target positioning method, system and computer equipment based on hierarchical class activation graph
A target positioning and hierarchical technology, applied in the field of deep learning, can solve problems such as inaccurate positioning ability, and achieve the effect of saving time and cost, reducing computational complexity, and achieving significant target positioning ability.
- Summary
- Abstract
- Description
- Claims
- Application Information
AI Technical Summary
Problems solved by technology
Method used
Image
Examples
Embodiment 1
[0047] A target positioning method based on a hierarchical class activation map of the present invention, the method includes inputting the image to be predicted into a convolutional hierarchical structure, extracting the hierarchical features of the image to be predicted, and generating a hierarchical class activation map of the image to be predicted ;Reserve some values in the hierarchical class activation map, and generate a bounding box that can predict the target to be tested;
[0048] Among them, such as figure 1 As shown, the generation of the hierarchical class activation map includes the following steps:
[0049] S1, Construct the convolutional hierarchical structure of the image to be predicted, including adding a layer custom convolutional layer;
[0050] S2, setting the step size and padding of the custom convolution layer added in S1;
[0051] S3. Superimpose the custom convolution layers corresponding to the convolution layer 4-3 and the convolution layer 4-...
Embodiment 2
[0057] This embodiment presents another embodiment of the present invention. In this embodiment, input the image to be tested into the model, calculate the loss function, and train the model until the loss function converges. Otherwise, use the gradient descent algorithm to update each parameter and continue to input Go to the model for training; when the model is trained, input the image to be tested, and extract the feature maps of convolutional layer 4-3, convolutional layer 4-4, convolutional layer 5-3, and convolutional layer 5-4, According to the formula (5), the saliency map I of the classification is determined A and I B ; and these two significant maps are superimposed to obtain a hierarchical class activation map; retain some values in the activation map, in this embodiment, select a value greater than 20% of the maximum activation value to retain; use it to generate a predicted bounding box.
[0058] Among them, the loss function may adopt several types of loss f...
Embodiment 4
[0076] This embodiment provides relevant descriptions of the target positioning system in the present invention;
[0077] The present invention also proposes a target positioning system based on a hierarchical class activation map, the system comprising:
[0078] An image acquisition module, configured to acquire an image to be predicted;
[0079] The hierarchical feature extraction module is used to extract the hierarchical features in the image to be predicted;
[0080] A hierarchical class activation map building block, used to construct a hierarchical class activation map from hierarchical features;
[0081] The predicted bounding box calculation module is used to predict the bounding box of the target to be tested in the image to be predicted according to the hierarchical class activation map;
[0082] Wherein, the hierarchical feature extraction module includes a VGG19 network structure, a self-defined convolutional layer unit, an overlay layer unit, and a pooling laye...
PUM
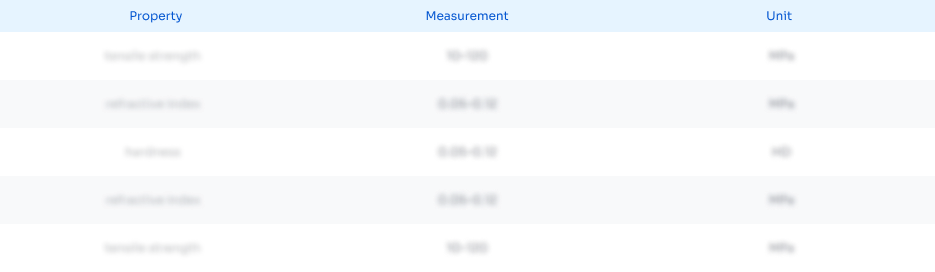
Abstract
Description
Claims
Application Information
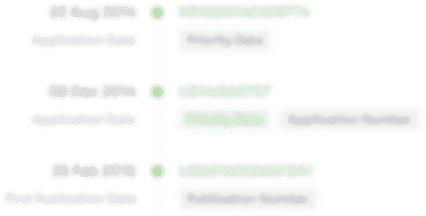
- R&D Engineer
- R&D Manager
- IP Professional
- Industry Leading Data Capabilities
- Powerful AI technology
- Patent DNA Extraction
Browse by: Latest US Patents, China's latest patents, Technical Efficacy Thesaurus, Application Domain, Technology Topic, Popular Technical Reports.
© 2024 PatSnap. All rights reserved.Legal|Privacy policy|Modern Slavery Act Transparency Statement|Sitemap|About US| Contact US: help@patsnap.com