A Differential Privacy High-Dimensional Data Publishing Protection Method Based on Principal Component Analysis Optimization
A principal component analysis, differential privacy technology, applied in digital data protection, electronic digital data processing, instruments, etc., can solve the problems of exponential growth of publishing space, dimensional disaster, inappropriate high-dimensional data publishing, etc. Disaster problems, effects of optimizing time and space
- Summary
- Abstract
- Description
- Claims
- Application Information
AI Technical Summary
Problems solved by technology
Method used
Image
Examples
Embodiment Construction
[0061] The present invention will be further described in detail below in conjunction with the accompanying drawings, so that those skilled in the art can implement it with reference to the description.
[0062] like figure 2 As shown, the method for publishing and protecting differentially private high-dimensional data based on principal component analysis optimization of the present invention proposes a PCAO_PPDP method for publishing high-dimensional data with differential privacy based on principal component analysis optimization, which utilizes principal component analysis based on information entropy The method reduces the dimensionality of the data, and uses the personalized Laplace mechanism to ensure that PCAO_PPDP meets the requirements of differential privacy. Theoretical analysis shows that the proposed PCAO_PPDP algorithm satisfies ε-differential privacy; the experimental results show that compared with the existing research work, the data utility of the data set g...
PUM
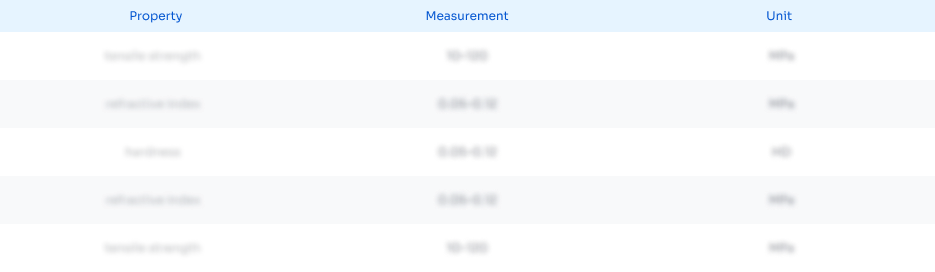
Abstract
Description
Claims
Application Information
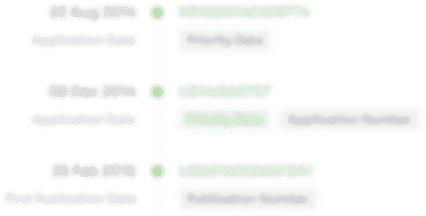
- R&D
- Intellectual Property
- Life Sciences
- Materials
- Tech Scout
- Unparalleled Data Quality
- Higher Quality Content
- 60% Fewer Hallucinations
Browse by: Latest US Patents, China's latest patents, Technical Efficacy Thesaurus, Application Domain, Technology Topic, Popular Technical Reports.
© 2025 PatSnap. All rights reserved.Legal|Privacy policy|Modern Slavery Act Transparency Statement|Sitemap|About US| Contact US: help@patsnap.com