Graph clustering method based on robust rank constraint sparse learning
A graph clustering and sparse technology, applied in the field of graph clustering, can solve problems such as poor robustness, and achieve the effect of improving robustness
- Summary
- Abstract
- Description
- Claims
- Application Information
AI Technical Summary
Problems solved by technology
Method used
Image
Examples
Embodiment Construction
[0023] refer to figure 1 . The specific steps of the graph clustering method based on robust rank-constrained sparse learning in the present invention are as follows:
[0024] Step 1. Learn the data similarity graph S through the sparse representation method, and at the same time combine L 2,1 Norm, in order to improve the quality of graph construction and reduce the impact of data noise and outliers. Specifically, X is the data, and the initial objective function is:
[0025] Step 2. Use the k-neighborhood method to construct the initial graph, and pass the regularization term Constrain the similarity graph S to be found in the neighborhood of the initial graph B, so that the learned similarity graph can accurately reflect the relationship between the data;
[0026] Step 3. Add the Laplacian rank constraint, that is, the Laplacian matrix L of S s The rank is constrained so that the rank is equal to the number of data points minus the number of connected regions in the...
PUM
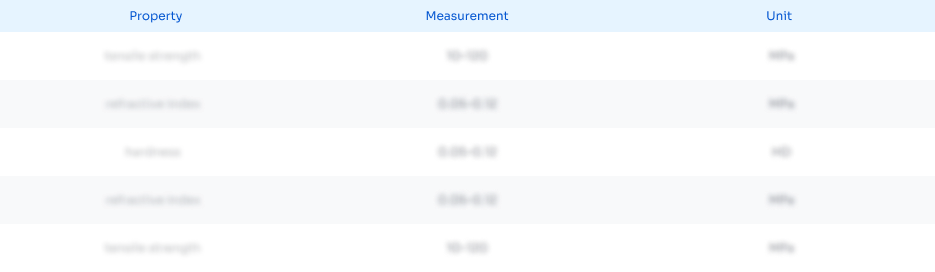
Abstract
Description
Claims
Application Information
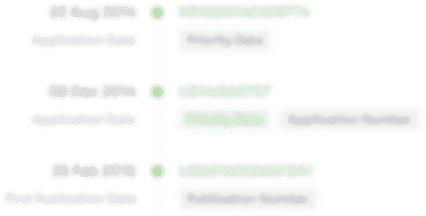
- R&D
- Intellectual Property
- Life Sciences
- Materials
- Tech Scout
- Unparalleled Data Quality
- Higher Quality Content
- 60% Fewer Hallucinations
Browse by: Latest US Patents, China's latest patents, Technical Efficacy Thesaurus, Application Domain, Technology Topic, Popular Technical Reports.
© 2025 PatSnap. All rights reserved.Legal|Privacy policy|Modern Slavery Act Transparency Statement|Sitemap|About US| Contact US: help@patsnap.com