Tool wear amount prediction method based on self-attention mechanism and depth learning
A technology of tool wear and deep learning, applied in the direction of neural learning methods, manufacturing tools, measuring/indicating equipment, etc., can solve problems such as the ability to process sequence data to be improved, and the prediction accuracy of tool wear is not high, so as to improve real-time prediction effect, Improve prediction effect, effect of improved ability
- Summary
- Abstract
- Description
- Claims
- Application Information
AI Technical Summary
Problems solved by technology
Method used
Image
Examples
specific Embodiment
[0041]The PHM (Fault Diagnosis and Health Management) Association is an internationally influential organization spanning academia and industry, dedicated to the research and application of the theory and method of "Fault Prediction and Health Management". The PHM Association was established in New York, USA in 2009, and holds the "PHM Association Data Competition" every year. This competition is one of the high-level similar competitions in the world. This example uses the competition data of the 2010 PHM Association Data Competition to verify the CNC machine tool wear prediction method proposed by the present invention.
[0042] The processing parameters of the CNC machine tools are: the spindle speed is 10400rpm, the feed rate in the x-axis direction is 1555mm / min, the radial cutting depth is 0.125mm, and the axial cutting depth is 0.2mm. The sensor arrangement is: as attached figure 2 As shown, the tool 2 is installed on the rotating spindle 1, the workpiece 3 is fixed o...
specific Embodiment approach
[0045] S1. Install dynamometers, acceleration sensors and acoustic sensors on the CNC machine table, fixtures and workpieces;
[0046] Install a dynamometer between the workpiece and the fixture, install three acceleration sensors in three directions of the workpiece, and install an acoustic sensor on the machine table;
[0047] S2. Carry out a milling experiment on the tool, and collect relevant processing test data through the sensor in S1;
[0048] The cutting force of the tool in the three coordinate directions of x, y and z of the CNC machine tool can be obtained through a dynamometer, and the vibration signals of the workpiece in the three coordinate directions of x, y and z of the CNC machine tool can be obtained through three acceleration sensors. Acoustic sensors can obtain sound signals during CNC machine tool processing. That is, through a dynamometer, three acceleration sensors and an acoustic sensor, a total of 7 different processing characteristic signals are ob...
PUM
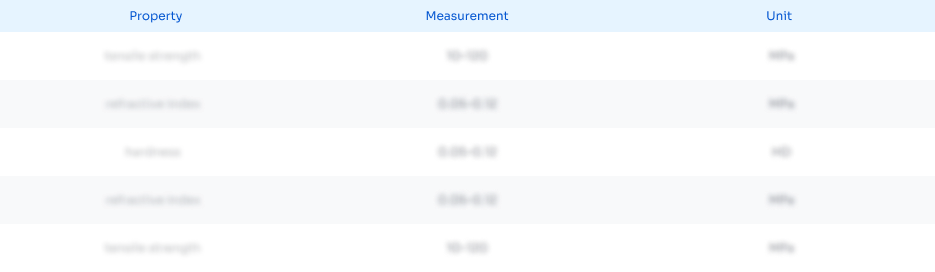
Abstract
Description
Claims
Application Information
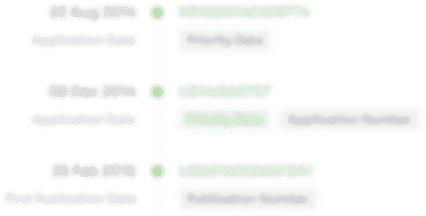
- R&D Engineer
- R&D Manager
- IP Professional
- Industry Leading Data Capabilities
- Powerful AI technology
- Patent DNA Extraction
Browse by: Latest US Patents, China's latest patents, Technical Efficacy Thesaurus, Application Domain, Technology Topic, Popular Technical Reports.
© 2024 PatSnap. All rights reserved.Legal|Privacy policy|Modern Slavery Act Transparency Statement|Sitemap|About US| Contact US: help@patsnap.com