Small sample target recognition method based on feature subspace generative adversarial network
A feature subspace and target recognition technology, applied in the field of deep learning, can solve problems such as the disadvantage of small sample target recognition
- Summary
- Abstract
- Description
- Claims
- Application Information
AI Technical Summary
Problems solved by technology
Method used
Image
Examples
Embodiment Construction
[0022] The present invention is further analyzed below in conjunction with specific examples.
[0023] Such as figure 1 As shown in , this experiment uses a set of collected ship target images as the training sample data set. The specific steps in the small sample target recognition task based on the feature subspace generation confrontation network are as follows:
[0024] Step (1), Obtain the features used to train the Generative Adversarial Network
[0025] 1.1 Use the classification network to perform rough training on image sample data.
[0026] Use the convolutional neural network to perform rough training on the image sample data. After training, the weight parameters and bias parameters are W and B respectively;
[0027] 1.2 Use W and B two sets of parameters to map the sample to the feature subspace to obtain feature data.
[0028] Assuming that there are n images in the sample data, the n image sample data is set A, and each image sample is A k (where 1
PUM
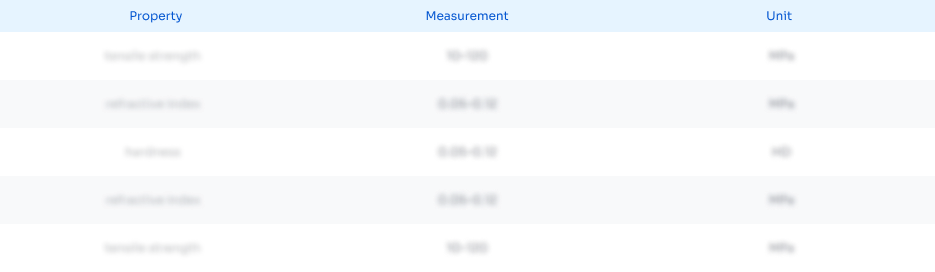
Abstract
Description
Claims
Application Information
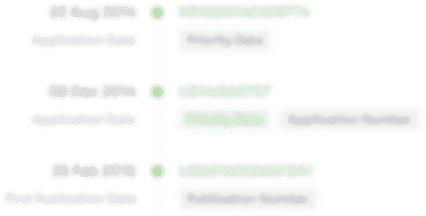
- R&D Engineer
- R&D Manager
- IP Professional
- Industry Leading Data Capabilities
- Powerful AI technology
- Patent DNA Extraction
Browse by: Latest US Patents, China's latest patents, Technical Efficacy Thesaurus, Application Domain, Technology Topic, Popular Technical Reports.
© 2024 PatSnap. All rights reserved.Legal|Privacy policy|Modern Slavery Act Transparency Statement|Sitemap|About US| Contact US: help@patsnap.com