Text coding representation method based on transformer model and multiple reference systems
A technology of transformer model and coding representation, applied in the field of machine understanding of natural language, can solve problems such as differences in understanding methods, and achieve the effect of solving difficult learning
- Summary
- Abstract
- Description
- Claims
- Application Information
AI Technical Summary
Problems solved by technology
Method used
Image
Examples
Embodiment Construction
[0048] In order to make the purpose, technical means and advantages of the present application clearer, the present application will be further described in detail below in conjunction with the accompanying drawings.
[0049] In the embodiment of the present invention, the sentences in the text are used as pre-training tasks. In view of the use of a single frame of reference to train the language model, the polysemy of words will interfere with the training effect. Therefore, at least one independent semantic meaning is set for each word. Representation, so that the most appropriate semantic representation can be derived in combination with the context to accurately train the contextualized semantic representation; in order to avoid the situation that at least one independent semantic cannot converge, the traction structure (semantic correlation) formed by weighting is used to achieve: When the actual number of semantic concepts (absolute number of semantic concepts) n of any w...
PUM
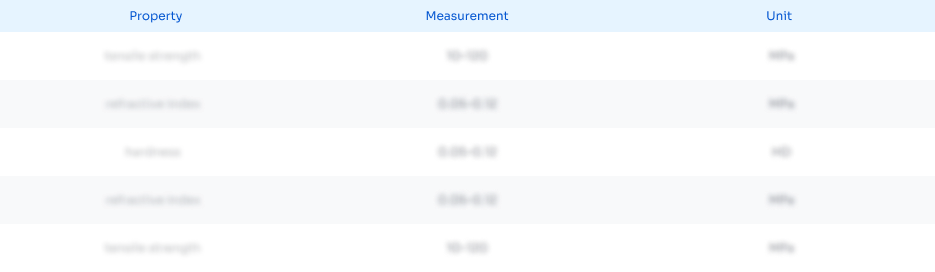
Abstract
Description
Claims
Application Information
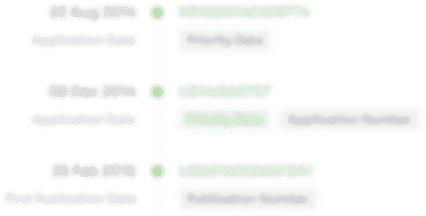
- R&D Engineer
- R&D Manager
- IP Professional
- Industry Leading Data Capabilities
- Powerful AI technology
- Patent DNA Extraction
Browse by: Latest US Patents, China's latest patents, Technical Efficacy Thesaurus, Application Domain, Technology Topic, Popular Technical Reports.
© 2024 PatSnap. All rights reserved.Legal|Privacy policy|Modern Slavery Act Transparency Statement|Sitemap|About US| Contact US: help@patsnap.com