Airborne flight parameter data health diagnosis method based on deep learning
A technology of deep learning and diagnostic methods, applied in the field of avionics, can solve problems such as difficult model training, failure to reflect aircraft characteristics in time, and rare applications in the aerospace field, so as to achieve the effect of reducing dependence and improving target recognition efficiency
- Summary
- Abstract
- Description
- Claims
- Application Information
AI Technical Summary
Problems solved by technology
Method used
Image
Examples
Embodiment Construction
[0029] The present invention will be described in further detail below in conjunction with accompanying drawing
[0030] Step 1: The avionics system parameters, lubricating oil system parameters, fire extinguishing system parameters, hydraulic system parameters, transmission system parameters, rotor parameters, fuel parameters, vibration parameters, inertial navigation parameters, electrical parameters, and A series of parameters such as cabin temperature, altitude and engine parameters are imported into the computer for data classification, analysis, initialization and preprocessing, and various data types are imported into a corresponding cache space on the PC to build a network training model.
[0031] Step 1.1: The flight data needs to be initialized before training. Usually, the Gaussian distribution initialization method or the random initialization method are used to initialize various types of data respectively.
[0032] Step 1.2: After the flight parameter data is ini...
PUM
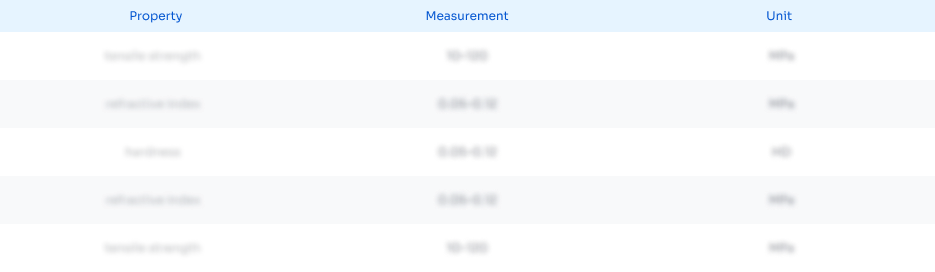
Abstract
Description
Claims
Application Information
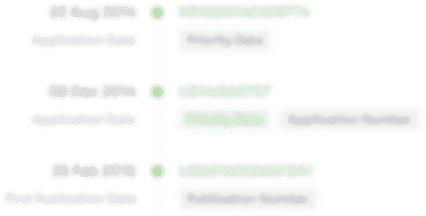
- R&D
- Intellectual Property
- Life Sciences
- Materials
- Tech Scout
- Unparalleled Data Quality
- Higher Quality Content
- 60% Fewer Hallucinations
Browse by: Latest US Patents, China's latest patents, Technical Efficacy Thesaurus, Application Domain, Technology Topic, Popular Technical Reports.
© 2025 PatSnap. All rights reserved.Legal|Privacy policy|Modern Slavery Act Transparency Statement|Sitemap|About US| Contact US: help@patsnap.com