Face attribute recognition method based on multi-task multi-label learning convolutional neural network
A convolutional neural network and attribute recognition technology, applied in neural learning methods, biological neural network models, neural architectures, etc., can solve problems such as affecting balance, unbalanced labels, confusing humans, etc., to improve accuracy and accuracy. Effect
- Summary
- Abstract
- Description
- Claims
- Application Information
AI Technical Summary
Problems solved by technology
Method used
Image
Examples
Embodiment Construction
[0041] The following examples will be described in detail in conjunction with the accompanying drawings and the method of the present invention. This example is implemented on the premise of the technical solution of the present invention, and the implementation and specific operation process are provided, but the protection scope of the present invention is not limited to the following the described embodiment.
[0042] see figure 1 , the embodiment of the present invention includes the following steps:
[0043] 1. Prepare the training sample set and verification sample. Using the open source python library requires face feature point labels, and the face attribute labels are the labels that come with the database.
[0044] A1. Obtain the annotations of face key point detection and face attribute recognition respectively;
[0045] A2. Integrate the annotations of face key point detection and face attribute recognition to form training and verification sample sets i=1,...,...
PUM
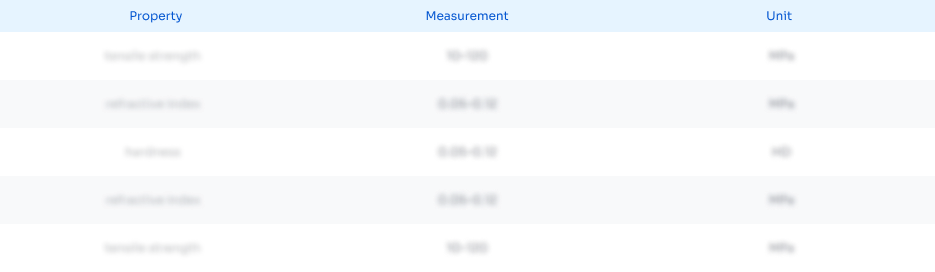
Abstract
Description
Claims
Application Information
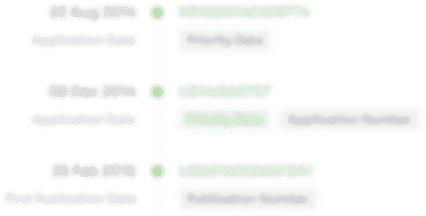
- R&D Engineer
- R&D Manager
- IP Professional
- Industry Leading Data Capabilities
- Powerful AI technology
- Patent DNA Extraction
Browse by: Latest US Patents, China's latest patents, Technical Efficacy Thesaurus, Application Domain, Technology Topic, Popular Technical Reports.
© 2024 PatSnap. All rights reserved.Legal|Privacy policy|Modern Slavery Act Transparency Statement|Sitemap|About US| Contact US: help@patsnap.com