A Weakly Supervised Semantic Segmentation Method and System Based on Graffiti
A semantic segmentation and weak supervision technology, applied in the field of machine learning and computer vision, can solve problems such as discontinuity, incoherence, and inconsistent segmentation results, and achieve the effect of improving performance
- Summary
- Abstract
- Description
- Claims
- Application Information
AI Technical Summary
Problems solved by technology
Method used
Image
Examples
Embodiment Construction
[0058] The present invention proposes an innovative boundary-aware guided model for scribble-based weakly supervised semantic segmentation tasks. The boundary-aware guidance model consists of two components: (1) The boundary correction network, which combines high-level semantic information and low-level edge / texture information at the same time, uses an iterative upsampling strategy instead of a rough direct 8x upsampling operation, which can generate fine feature maps. (2) Boundary regression network, which can guide the network to obtain clear boundaries between different semantic regions.
[0059] In order to make the above-mentioned features and effects of the present invention more clear and understandable, the following specific examples are given together with the accompanying drawings for detailed description as follows.
[0060] In order to solve the above two problems, the present invention fully excavates the high-level semantic features and low-level high-resoluti...
PUM
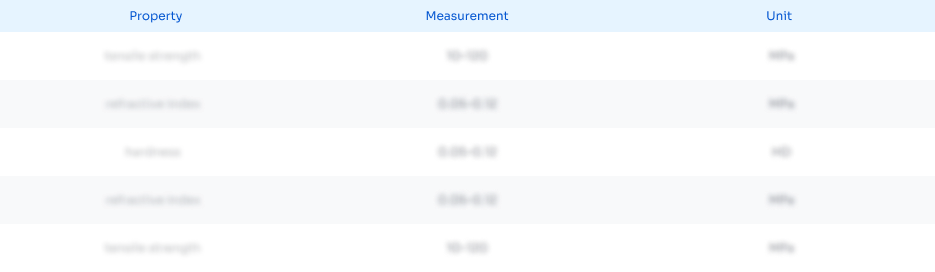
Abstract
Description
Claims
Application Information
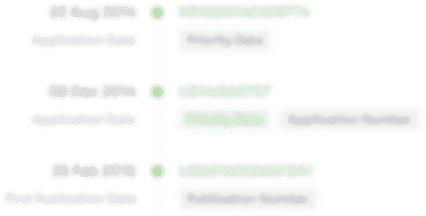
- Generate Ideas
- Intellectual Property
- Life Sciences
- Materials
- Tech Scout
- Unparalleled Data Quality
- Higher Quality Content
- 60% Fewer Hallucinations
Browse by: Latest US Patents, China's latest patents, Technical Efficacy Thesaurus, Application Domain, Technology Topic, Popular Technical Reports.
© 2025 PatSnap. All rights reserved.Legal|Privacy policy|Modern Slavery Act Transparency Statement|Sitemap|About US| Contact US: help@patsnap.com