Method of establishing scma codec model based on denoising autoencoder
A self-encoder and codec technology, applied in the field of SCMA codec model establishment, can solve the problems of slow convergence speed and long training time of deep neural network
- Summary
- Abstract
- Description
- Claims
- Application Information
AI Technical Summary
Problems solved by technology
Method used
Image
Examples
Embodiment 1
[0113] Take the case of 6 users and 4 resource blocks as an example.
[0114] Such as figure 1 As shown, based on the idea of denoising self-encoder, a certain proportion of random noise is added to the input data, and the SCMA encoder maps the user's original input data into codewords; the encoded codewords are superimposed on the resource blocks in a non-orthogonal manner Transmission, affected by the channel during transmission, the signal is superimposed with noise; a SCMA decoder based on a fully connected neural network is established at the receiving end, and the original input information of all users is decoded according to the input codeword; the above SCMA codec can As a complete noise reduction autoencoder structure, superimpose a fixed size channel noise to train the above SCMA codec model based on noise reduction autoencoder; test the BER of the above SCMA codec model based on noise reduction autoencoder performance. Compared with the traditional SCMA system,...
PUM
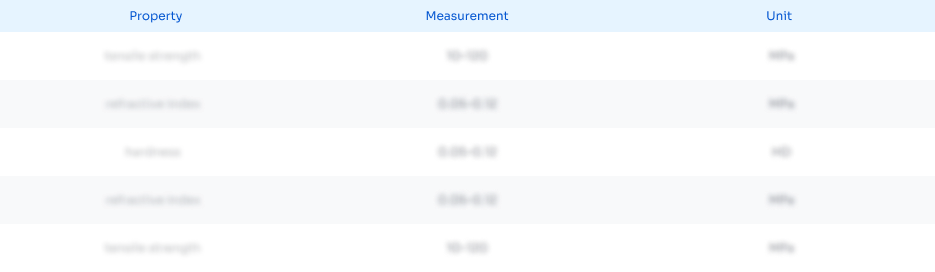
Abstract
Description
Claims
Application Information
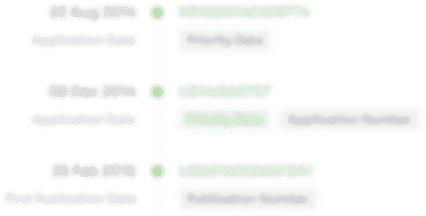
- R&D Engineer
- R&D Manager
- IP Professional
- Industry Leading Data Capabilities
- Powerful AI technology
- Patent DNA Extraction
Browse by: Latest US Patents, China's latest patents, Technical Efficacy Thesaurus, Application Domain, Technology Topic, Popular Technical Reports.
© 2024 PatSnap. All rights reserved.Legal|Privacy policy|Modern Slavery Act Transparency Statement|Sitemap|About US| Contact US: help@patsnap.com