Zero-sample image classification method based on variational self-coding adversarial network
A technology of sample images and classification methods, which is applied to computer parts, instruments, characters and pattern recognition, etc., and can solve problems such as easy distortion of visual features
- Summary
- Abstract
- Description
- Claims
- Application Information
AI Technical Summary
Problems solved by technology
Method used
Image
Examples
Embodiment Construction
[0043] A zero-shot image classification method based on variational self-encoding confrontation network of the present invention will be described in detail below with reference to the embodiments and drawings.
[0044] A zero-shot image classification method based on variational self-encoded adversarial networks of the present invention assumes that while using semantic features to generate visual features, the two-way alignment between semantic features and visual features is considered. On the basis of using two VAEs for visual and semantic modalities respectively, a discriminator is introduced to achieve bidirectional alignment of visual semantic features and make VAE generate pseudo visual features closer to real features.
[0045] A zero-shot image classification method based on variational autoencoder confrontation network of the present invention is to construct two variational autoencoders (VAE) of visual modality and semantic modality and use visual features and seman...
PUM
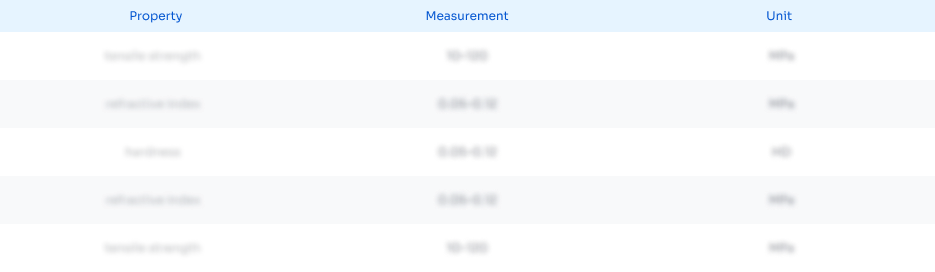
Abstract
Description
Claims
Application Information
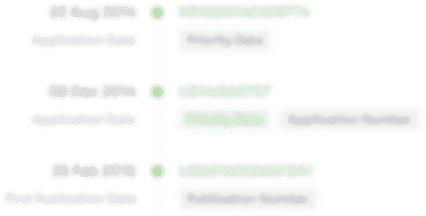
- R&D
- Intellectual Property
- Life Sciences
- Materials
- Tech Scout
- Unparalleled Data Quality
- Higher Quality Content
- 60% Fewer Hallucinations
Browse by: Latest US Patents, China's latest patents, Technical Efficacy Thesaurus, Application Domain, Technology Topic, Popular Technical Reports.
© 2025 PatSnap. All rights reserved.Legal|Privacy policy|Modern Slavery Act Transparency Statement|Sitemap|About US| Contact US: help@patsnap.com