Data flow classification algorithm based on AAE-DWMAL-LearnNSE (Automatic Assisted Engineering-Discrete Wavelength Multiple Input Multiple Output-LearnNSE)
A classification algorithm and data flow technology, applied to other database clustering/classification, other database retrieval, etc., can solve the problems of classification accuracy impact, distribution, etc., achieve accurate classification features, save storage space, and reduce model construction time Effect
- Summary
- Abstract
- Description
- Claims
- Application Information
AI Technical Summary
Problems solved by technology
Method used
Image
Examples
Embodiment Construction
[0042] specific implementation plan
[0043] The present invention will be described in further detail below through examples of implementation.
[0044] The data set selected in this implementation case has a total of 6 sets of samples, including 50,000 sets of moving Gaussian, ocean, hyperplane, chessboard, electric power and weather data, and 40,000 samples are extracted from each of the 6 sets of data by random sampling as a training set , and the remaining 10,000 are used as the test set.
[0045] The overall flow of the data stream classification algorithm provided by the present invention is as follows: figure 1 As shown, the specific steps are as follows:
[0046] (1) Update each classifier Weights
[0047] First, calculate the classification error rate ε of each base classifier, and set the initial value of the weight ω of each base classifier according to the classification error rate, as shown in the following formula:
[0048]
[0049] In the formula, j=...
PUM
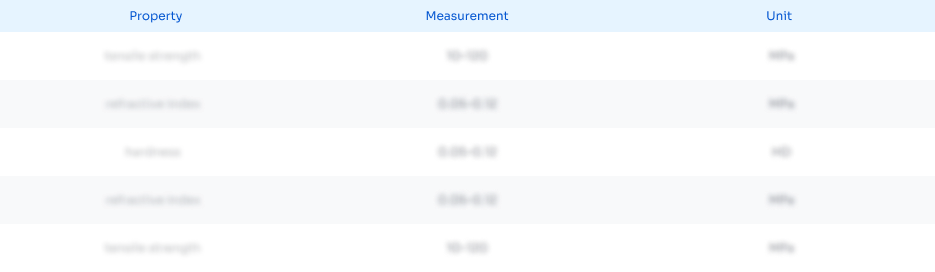
Abstract
Description
Claims
Application Information
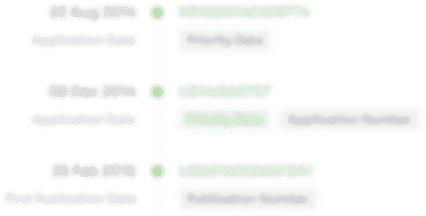
- R&D
- Intellectual Property
- Life Sciences
- Materials
- Tech Scout
- Unparalleled Data Quality
- Higher Quality Content
- 60% Fewer Hallucinations
Browse by: Latest US Patents, China's latest patents, Technical Efficacy Thesaurus, Application Domain, Technology Topic, Popular Technical Reports.
© 2025 PatSnap. All rights reserved.Legal|Privacy policy|Modern Slavery Act Transparency Statement|Sitemap|About US| Contact US: help@patsnap.com