Cell load prediction method based on improved clustering and long-term and short-term memory deep learning
A long-short-term memory and deep learning technology, which is applied in the field of auxiliary construction of distribution network industry expansion and installation, can solve problems such as large errors in artificial estimation, unreasonable construction planning of station areas, and few impact factors
- Summary
- Abstract
- Description
- Claims
- Application Information
AI Technical Summary
Problems solved by technology
Method used
Image
Examples
Embodiment Construction
[0070] The application will be further described below in conjunction with the accompanying drawings. The following examples are only used to illustrate the technical solutions of the present invention more clearly, but not to limit the protection scope of the present application. ,
[0071] The present invention proposes a community load prediction method based on improved clustering and long-term and short-term memory deep learning: according to the impact factors of different types of residential areas, the housing types are divided, and corresponding prediction models are established for each type of residential area to obtain the predicted load value , Realize the reasonable planning of the transformer in the Taiwan area.
[0072] In the embodiment, the total number of residential quarter samples is about 51, and the impact factor is 7, which means that there are 7 input feature dimensions.
[0073] A community load prediction method based on improved clustering and lon...
PUM
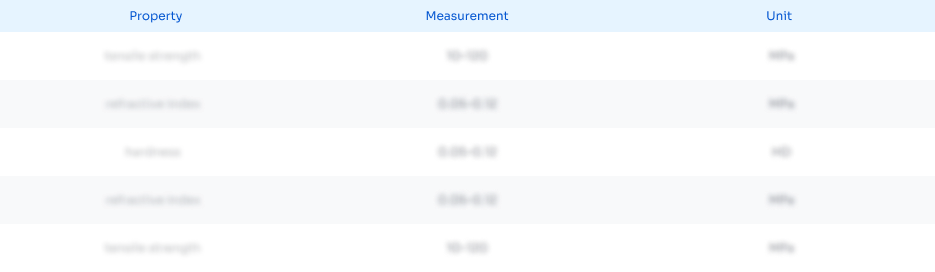
Abstract
Description
Claims
Application Information
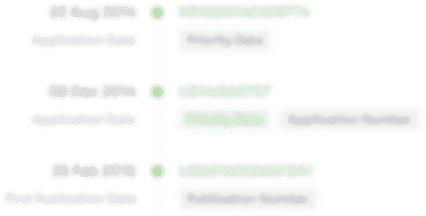
- R&D
- Intellectual Property
- Life Sciences
- Materials
- Tech Scout
- Unparalleled Data Quality
- Higher Quality Content
- 60% Fewer Hallucinations
Browse by: Latest US Patents, China's latest patents, Technical Efficacy Thesaurus, Application Domain, Technology Topic, Popular Technical Reports.
© 2025 PatSnap. All rights reserved.Legal|Privacy policy|Modern Slavery Act Transparency Statement|Sitemap|About US| Contact US: help@patsnap.com