Intersection recognition method based on GoogLeNet neural network
An intersection and neural network technology, applied in the direction of biological neural network model, neural architecture, character and pattern recognition, etc., can solve the problem of inability to effectively distinguish complex road intersection structures, reduce the convenience of complex intersection identification, and complex intersection deviation diagrams. It can achieve the effect of fast and high-quality positioning, abundant samples, and large total volume.
- Summary
- Abstract
- Description
- Claims
- Application Information
AI Technical Summary
Problems solved by technology
Method used
Image
Examples
Embodiment
[0041] Selection of national samples:
[0042] The road network data of 39 major cities across the country: Beijing, Shanghai, Guangzhou, Shenzhen, Hangzhou, Wuhan, Xiamen, Zhuhai, etc. are selected as the sampling source for samples of complex intersections, such as figure 2 , 3 , As shown in 4;
[0043] Determine the location and spatial scope of complex intersections by constructing a Delaunay triangle network:
[0044] Step 1: In the road network vector data, construct the node-arc topology, and identify the nodes associated with three or more arcs, such as Figure 5 (A) as shown;
[0045] Step 2: Based on the nodes in step 1, build a Delaunay triangulation network, such as Figure 5 (B) Shown;
[0046] Step 3: Using each node as a statistical unit, identify the length of each side associated with it in the Delaunay triangulation network, and calculate the local average length and length standard deviation;
[0047] Step 4: Repeat step 3 until all nodes are counted, calculate the av...
PUM
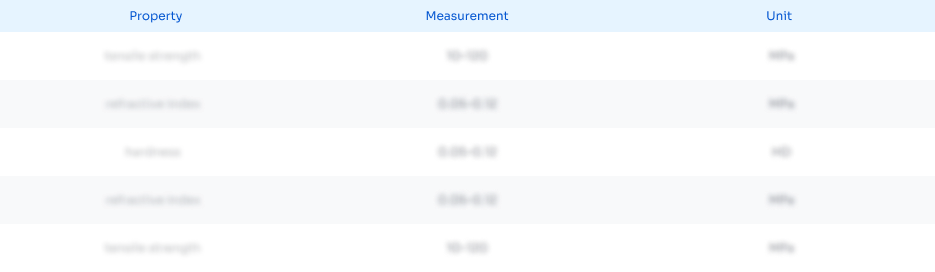
Abstract
Description
Claims
Application Information
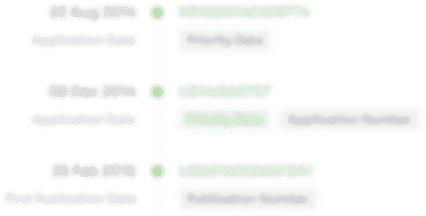
- R&D Engineer
- R&D Manager
- IP Professional
- Industry Leading Data Capabilities
- Powerful AI technology
- Patent DNA Extraction
Browse by: Latest US Patents, China's latest patents, Technical Efficacy Thesaurus, Application Domain, Technology Topic, Popular Technical Reports.
© 2024 PatSnap. All rights reserved.Legal|Privacy policy|Modern Slavery Act Transparency Statement|Sitemap|About US| Contact US: help@patsnap.com